AI Proof of Concept: Benefits, Stages, Challenges, and More
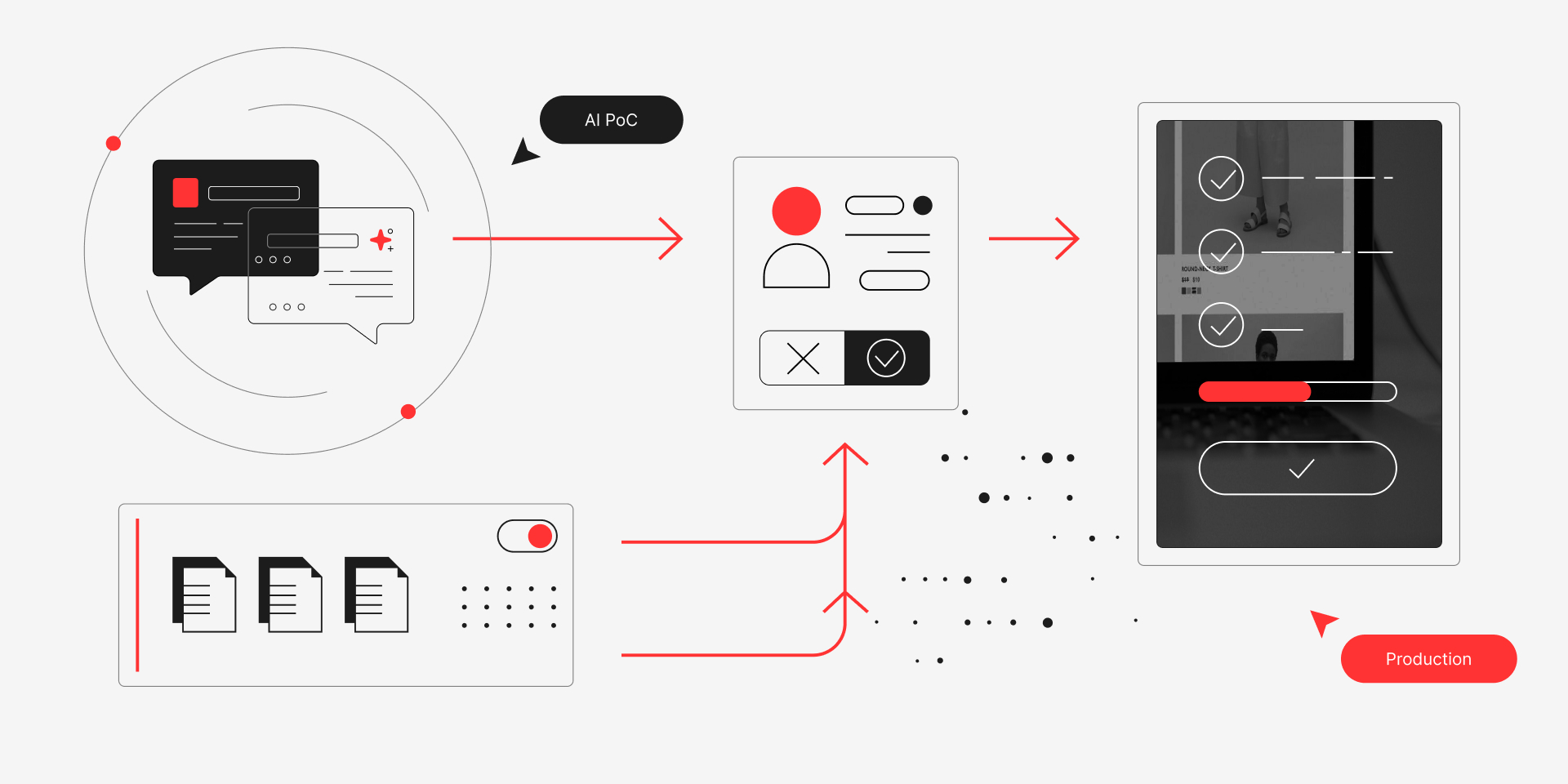
The impact of an AI breakthrough we are currently living through cannot be overstated. Quickly and confidently, Artificial Intelligence has made its way to nearly every aspect of our lives, from healthcare to communications. With plenty of AI-driven solutions out there, the market is still hungry for more, inspiring individuals, startups, and enterprises to create their own products.
At the same time, starting a full-scale development process right away may not be the wisest decision, given that it’s nearly impossible to know for a fact whether a product idea would work or not. This is why it’s now common for companies to start with an AI Proof of Concept. An AI PoC helps determine the potential of a product idea and pivot if necessary while keeping the resource use to a minimum. Find out why do an AI PoC in the first place, how to do it the right way, and what kind of challenges to be aware of from today’s guide.
What Is an AI Proof of Concept?
An AI proof of concept (PoC) is a small-scale, experimental project that deals with AI implementation. The purpose of an artificial intelligence POC is to validate the functionality, feasibility, and potential value of an artificial intelligence solution for solving a specific business problem.
It serves to demonstrate whether the AI endeavor can achieve the desired outcomes under controlled conditions, allowing stakeholders to evaluate its technical viability, potential impact on the business operations, alignment with business goals, and return on investment of time and resources before proceeding with full-scale development or deployment.
“Our early AI PoC projects were important for helping our clients see the potential of AI and how it could fit into their businesses. Over time, we’ve changed our approach based on what we’ve learned from each project. Now, we focus more on making sure our PoCs can either fit smoothly with the client’s existing products or work on their own. This means our solutions are less disruptive and more immediately useful. We also concentrate on the most important tasks our clients need help with, ensuring that our PoCs address real problems effectively.”
Yuriy Khoma, Co-Founder and CEO, UnidataLab
AI PoC vs. AI Prototype: Are They the Same Thing?
An AI PoC and an AI prototype have similar business objectives: most importantly, to help the stakeholders decide whether to proceed with the full-scale development project. Still, there are some important distinctions between the two, as well as the most suitable uses for each one. Here are a few factors to help you better understand how they are different.
Objective
An AI PoC tests whether an AI solution is feasible and valuable, while an AI Prototype focuses on demonstrating its functionality, usability, and workflows in practice.
Scope
AI PoCs have a narrow focus on feasibility using minimal resources. AI prototypes simulate real-world conditions with broader features like workflows, interfaces, and system integrations.
Output
An AI PoC produces evidence of feasibility and value, often in a report. An AI prototype delivers a working model showcasing how the AI solution would operate.
Development effort
AI PoCs require minimal development effort to prove feasibility. AI prototypes demand more effort, incorporating functionality, user interfaces, and basic integrations to simulate the solution.
Use cases
AI PoCs validate new AI concepts in uncertain areas. AI Prototypes refine workflows, test usability, and gather feedback before scaling the solution to full production.
Have a Product Idea You Want to Test Out? Count on us for the perfect execution.
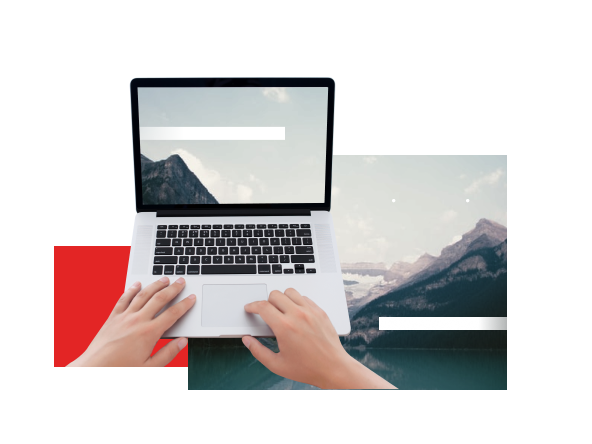
When to Use AI PoCs and What Can You Gain from AI Proof of Concept?
Undertaking a new AI initiative can seem like a daunting task: no matter how common AI technologies are becoming, the decision to deploy a custom AI solution is a big step to take. A PoC serves as the most effective way to validate the potential impact of the AI solution and whether a full-scale project is the right step to take. Here is where AI PoC development is the perfect fit for your project.
“AI PoC is well-suited for product companies looking to widen their services’ capabilities and functionality. Such companies have usually managed to implement crucial processes and services, and are now interested in improving them.”
Vladyslav Lisovskyi, Senior Data Science Engineer, Amazinum
Evaluating the Feasibility of AI Solutions
When businesses are unsure if AI can effectively address a specific problem or align with their goals, a PoC helps validate the concept before investing significant resources.
Exploring New Use Cases
When implementing AI in untested areas, such as automating unique processes or entering new markets, a PoC assesses its potential without committing to full-scale deployment.
Assessing Technical Viability
For businesses venturing into advanced AI techniques like machine learning or natural language processing, a PoC ensures the technology can function within their existing infrastructure.
Minimizing Risks in High-Stakes Projects
In industries like healthcare, finance, or manufacturing, where errors have serious consequences, a PoC mitigates risks by thoroughly testing the solution beforehand.
Securing Stakeholder Buy-In
When decision-makers require proof of value, an AI PoC provides tangible results to demonstrate the potential ROI and gain support for larger investments.
Optimizing Costs and Resources
If businesses have limited budgets or in-house expertise, a PoC ensures resources are directed toward solutions that are likely to succeed.
Preparing for Scalability
Before committing to full-scale AI deployment, a PoC allows businesses to identify potential challenges, refine models, and ensure scalability for long-term use.
Experimenting with Emerging Technologies
For organizations adopting cutting-edge AI tools or frameworks, a PoC provides a safe environment to test their applicability and effectiveness.
“I’ve worked on multiple AI Proofs of Concept (PoCs) across several verticals — ranging from manufacturing to finance, healthcare, and non-profits. Early on, I focused heavily on the technical side: building models, experimenting with data, and showcasing what AI could do. Over time, however, I realized that the people factor is just as critical as the technology — if not more so. If we had a chance to start over on those early projects, there are some things we would do differently — namely, establish a feedback loop as early as possible, prioritize stakeholder alignment, and focus on the human side of AI adoption.”
Matt Kurleto, CEO, Neoteric
Key Use Cases of AI Proof of Concept
Artificial intelligence has countless uses, and their number only grows by the year. No matter what your organization deals with, there is a specific AI use that can help you move it forward, refine your customer experience, and make informed decisions about the direction and future of your business. Here are 10 real-world use cases of implementing AI tools with the help of a PoC:
- Customer service automation. Here, you can test AI chatbots or virtual assistants to handle common customer queries, reduce response times, and improve customer satisfaction while lowering operational costs.
- Fraud detection. This use case allows you to validate AI models to identify fraudulent transactions or activities in real time, helping financial institutions enhance security and reduce losses.
- Predictive maintenance. This enables you to assess the ability of AI to analyze equipment data, predict failures, and schedule maintenance proactively, minimizing downtime and costs in manufacturing and logistics.
- Personalized marketing. Here you can test AI algorithms that deliver tailored recommendations and targeted advertising, boosting customer engagement and sales in eCommerce and retail.
- Healthcare diagnostics. This allows you to evaluate AI’s capability to analyze medical images or patient data for faster and more accurate diagnoses, improving treatment outcomes.
- Supply chain optimization. In this use case, you can assess AI’s potential to forecast demand, optimize inventory, and improve logistics, driving efficiency and cost savings in supply chain operations.
- Natural Language Processing. This allows you to test AI’s ability to extract insights from unstructured text, such as legal documents or customer feedback, to streamline decision-making and enhance productivity.
- Image recognition. Here, you can validate AI’s performance in detecting objects, people, or patterns in images for use cases like quality control, security surveillance, or product categorization.
- Financial forecasting. This use case enables you to assess AI’s ability to predict revenue trends, market shifts, or investment risks, enabling more informed decision-making in financial planning.
- Talent acquisition. This gives you an ability to test AI-powered recruitment tools to screen resumes, identify top candidates, and reduce hiring time, improving HR efficiency.
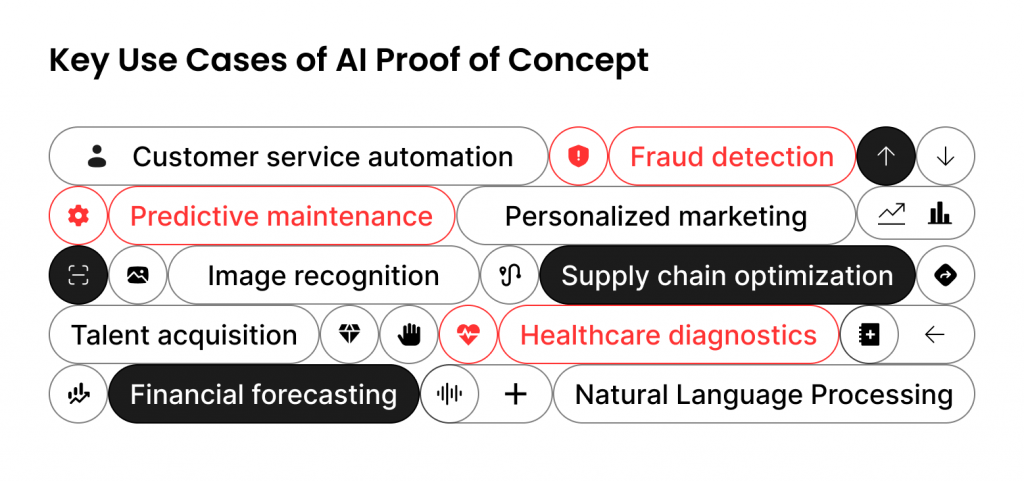
“AI PoCs can be beneficial for many different industries, especially those that deal with large amounts of data or rely heavily on customer interactions. A few areas where we’ve seen AI PoCs make a real difference include healthcare, where AI not only improves patient satisfaction but also reduces the administrative workload for staff, EdTech, where AI helps increase course completion rates and provides students with the guidance they need to succeed, eCommerce, where the use of AI leads to higher customer satisfaction and reduced the workload on support teams, and fintech & banking, where AI helps institutions enhance security and improve their interactions with customers.
Yuriy Khoma, Co-Founder and CEO, UnidataLab
Why Businesses Need AI PoC: The Biggest Benefits AI PoCs Offer
For businesses, scaling an unvalidated AI project can lead to wasted resources, unmet expectations, or even reputational damage if the solution underperforms. A PoC provides clarity and confidence, ensuring that investments in AI are backed by measurable results and clear insights into potential benefits and challenges.
Early Risk Mitigation
An AI PoC identifies technical limitations, business misalignments, and potential risks at the initial stage. Addressing these early helps avoid costly errors during full-scale development and ensures the project stays on track.
Focused Resource Allocation
A PoC ensures resources such as budget, time, and talent are directed toward solutions with proven potential, preventing waste on ideas that may not deliver desired outcomes or align with business goals.
Clear Success Metrics
Developing an AI PoC allows businesses to define measurable success criteria specific to their challenges, ensuring the project’s objectives and outcomes are clearly understood and actionable from the start.
Faster Iteration Cycles
The small-scale nature of a PoC facilitates rapid testing and iteration. Businesses can experiment with different models, algorithms, or approaches, quickly refining ideas and finding the optimal solution before scaling up.
Innovation Without Commitment
An AI PoC provides a safe, low-cost environment for businesses to explore cutting-edge AI technologies. This fosters innovation and experimentation without the pressure of committing to a full-scale, potentially risky implementation.
Improved Decision-Making
Insights gathered from the PoC offer valuable data for evaluating the solution’s potential. This empowers decision-makers to make informed choices about whether to proceed, pivot, or abandon a project before incurring significant expenses.
Enhanced Collaboration
AI PoCs encourage collaboration between technical teams, business leaders, and stakeholders. By aligning expectations early in the process, the PoC fosters a shared understanding of project goals, challenges, and opportunities.
Strengthened Stakeholder Confidence
Delivering tangible results through a PoC reassures stakeholders about the solution’s feasibility and value. This builds trust and secures buy-in for the next stages of AI development and deployment.
Competitive Advantage
A PoC accelerates the validation and adoption of innovative AI solutions. Businesses can implement successful AI concepts faster than competitors, gaining an edge in efficiency, customer experience, or market differentiation.
Scalability Testing
A PoC tests whether an AI solution can scale effectively with larger datasets, user bases, or infrastructure demands. This ensures the system is robust and ready for full-scale deployment without unforeseen bottlenecks.
Let’s turn your AI idea into a profitable product.
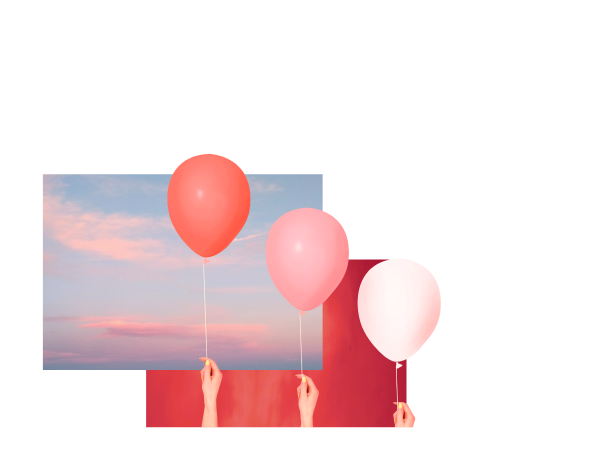
How to Develop a Proof of Concept in AI: 5 Steps for Building an AI Solution PoC
There is a lot riding on the development phase of an AI PoC, from the solution’s performance under different conditions to how well it’s eventually received by the intended audience. Here are the five steps required to develop and deploy AI solutions.
1. Define the Problem and Objectives
A successful AI PoC begins with a clear understanding of the business problem and what you aim to achieve. It’s important to not just discuss valuable information within the development team, but also involve other departments, as well as potential users who may provide priceless insights on what they actually want to get from the solution. This stage of the process typically includes the following activities:
- Identify the problem. Pinpoint a specific issue where AI could provide measurable value (for example, automating manual tasks or improving predictions).
- Set objectives. Define what success looks like using measurable KPIs. The examples of KPIs include accuracy rates, cost savings, or improved processing speed.
- Narrow the scope. Avoid tackling multiple problems at once. An effective AI development approach is about focusing on a single use case to reduce complexity and increase the chances of success.
- Align stakeholders. Make sure that technical teams, business executives, and decision-makers are on the same page about goals and expected outcomes.
“The PoC is mostly about the phase of discovery, so the most important thing is clearing the requirements and expectations of the project. Most of the time we aim to get answers to the following questions:
– What is the client’s domain? What does the business need?
– What problem does the client want to solve?
– What is the available data?
– What is the deliverable?
We can build a final proposal with a clear roadmap based on the response. Also, it’s important to stay focused during the PoC stage — it should be real and concise without overengineering and grand plans.”
Vladyslav Lisovskyi, Senior Data Science Engineer, Amazinum
2. Prepare the Data
Data is the foundation of any AI solution, and its quality directly impacts the PoC’s success. Here is how to manage the data if you want to build AI PoC that genuinely resonates with the users:
- Identify data sources. Determine where relevant data resides, whether it’s internal systems, third-party sources, or public datasets.
- Clean and preprocess data. Remove errors, duplicates, and inconsistencies. Ensure the data is structured, normalized, and ready for analysis.
- Ensure data representativeness. Verify that the dataset reflects the diversity and complexity of real-world scenarios to avoid biases.
- Address data gaps. If the data is insufficient, consider generating synthetic data, collecting additional data, or using transfer learning with pre-trained models.
3. Select the Right Tools and Approach
Choosing the right tools and methods is critical for building an effective PoC in any tech field, not to mention something as innovative and technically complex as an AI system. Here is how to make your AI idea work through the use of the right tools and frameworks:
- Pick the algorithm. Select AI techniques suited to the problem, such as machine learning, deep learning, or natural language processing.
- Choose tools and platforms. Opt for user-friendly frameworks like TensorFlow, PyTorch, and Scikit-learn, or AI platforms like Google Cloud AI or AWS SageMaker to accelerate development.
- Leverage pre-built solutions, Consider using APIs or pre-trained models to save time and resources for standard AI tasks like image recognition or sentiment analysis.
- Plan for scalability. Design the proposed AI solution with future expansion in mind by using flexible and modular tools.
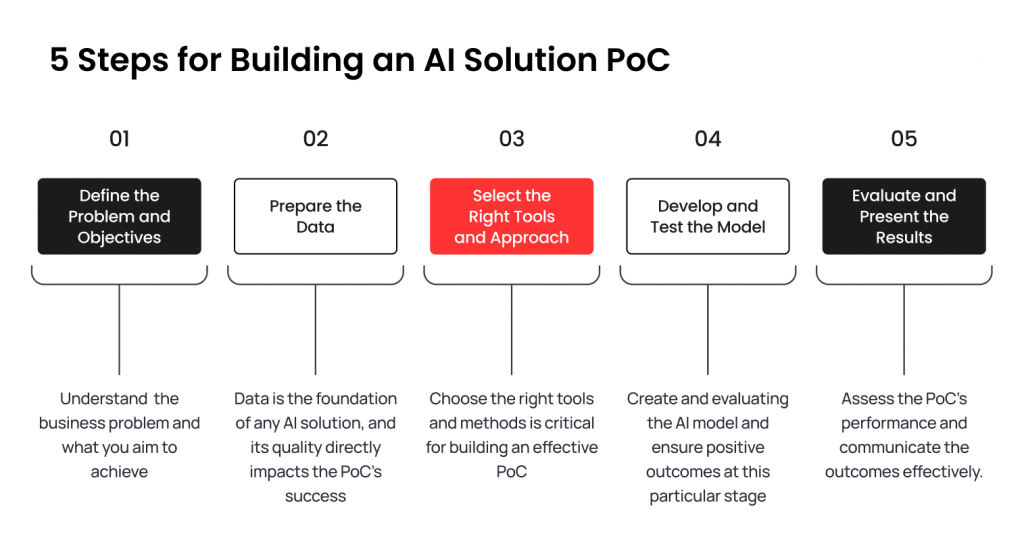
4. Develop and Test the Model
This step involves creating and evaluating the AI model. This is the most crucial stage in the AI PoC process, and although the previous steps also contribute greatly to a successful PoC project, there are a few ways to ensure positive outcomes at this particular stage:
- Build a simple model. Focus on core functionalities rather than a full-featured system. For example, prioritize testing the model’s ability to process data or make predictions.
- Train and fine-tune. Use your prepared data to train the AI model, refining parameters to improve performance.
- Test with real-world scenarios. Simulate practical use cases to see how the model performs under relevant conditions.
- Document your findings. Record model accuracy, limitations, and unexpected behaviors for analysis and improvement.
“The key to delivering functional and manageable AI PoC solutions is to provide a straightforward web app that demonstrates the key features you need. This mlevant regulations and ethical guakes it easy for clients to see how the AI solution works in practice. It’s also a good idea to include a user-friendly admin panel that allows clients to manage and monitor the AI solution without needing technical expertise.”
Yuriy Khoma, Co-Founder and CEO, UnidataLab
5. Evaluate and Present the Results
Finally, assess the PoC’s performance and communicate the outcomes effectively. Here is how to prepare for this step and manage it to get people on board with the product idea:
- Compare outcomes to KPIs. Evaluate the AI model’s performance against the predefined success metrics to determine feasibility and value.
- Create a presentation. Use dashboards, charts, or demos to visualize results in a way that stakeholders can easily understand.
- Identify the next steps. Provide recommendations for scaling the solution, revising the approach, or halting further development.
- Gather feedback. Engage stakeholders to understand their perspectives and refine the project based on their input.
Common Challenges of AI Development at the PoC Stage
The many AI PoC benefits are hard to argue with: the ability to test out an AI solution before investing and to make informed decisions about scaling or pivoting the product is invaluable in a rapidly changing tech world. At the same time, the process of developing an AI PoC might run into certain difficulties, so the more you know about those potential challenges, the better you are going to be equipped against them. Here are the 10 most common complications of developing an AI Proof of Concept and how to deal with them.
Lack of Clear Objectives
Without defined goals, a PoC may fail to deliver actionable insights and lead to unclear or irrelevant outcomes. This often results in wasted resources and stakeholder frustration.
How to mitigate: Establish specific, measurable objectives and KPIs aligned with business priorities before starting development.
Insufficient Data Quality or Quantity
AI models require clean, representative data to perform well. Poor-quality data can lead to biased, inaccurate results, while insufficient data may prevent the model from learning effectively.
How to mitigate: Conduct data audits, clean datasets, and, if necessary, generate synthetic data or gather additional real-world samples.
Misalignment Between Teams
Technical and business teams often have differing expectations and priorities, leading to poor communication and mismatched outcomes that fail to meet organizational goals.
How to mitigate: Foster collaboration through cross-functional workshops, ensuring all stakeholders understand the PoC’s goals, scope, and constraints.
Overly Ambitious Scope
Trying to tackle too many objectives can lead to delays, complexity, and failure to achieve meaningful insights. A broad scope often overstretches resources.
How to mitigate: Keep the PoC scope narrow and focus on testing one or two critical aspects of the AI solution.
Resource Constraints
Limited budget, time, or expertise can hinder progress and delay the PoC, especially for small or under-resourced teams.
How to mitigate: Prioritize minimal viable functionality, use pre-trained models or tools, and consider outsourcing or partnering with AI specialists.
Difficulty Demonstrating Value
Stakeholders may struggle to see the PoC’s potential benefits if the results are overly technical or disconnected from business metrics. This can undermine buy-in.
How to mitigate: Tie PoC outcomes to business metrics and present results in clear, stakeholder-friendly formats like dashboards or visual reports.
Technical Integration Issues
Integrating AI models with existing systems can be challenging due to compatibility issues, legacy systems, or infrastructure limitations. This often delays deployment.
How to mitigate: Test integration in a controlled environment and collaborate with IT teams to ensure compatibility and smooth deployment.
Unrealistic Expectations
Stakeholders may expect production-ready results from a PoC, leading to disappointment when the solution remains a proof-of-concept rather than a polished product.
How to mitigate: Communicate that a PoC is a feasibility test, not a final product, and set realistic expectations about its capabilities.
Scalability Concerns
PoC solutions may not translate well to full-scale deployment due to limitations in design, performance, or infrastructure readiness.
How to mitigate: Design the PoC with scalability in mind, using modular approaches and infrastructure that can support growth.
Ethical and Compliance Challenges
AI PoCs might inadvertently violate data privacy, intellectual property, or regulatory standards, exposing businesses to legal risks and reputational damage.
How to mitigate: Involve legal and compliance teams early, ensuring all datasets and algorithms adhere to relevant regulations and ethical guidelines.
Your product idea + Our expert-level execution = Market-disrupting solution
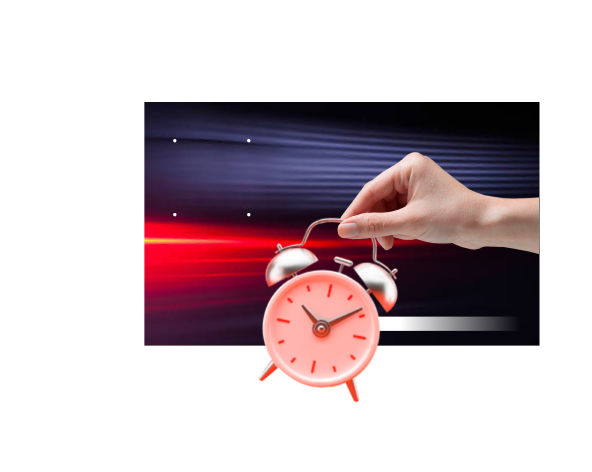
How to Maximize the Success of an AI PoC Development
All the innovation and technical impact that an AI PoC project can deliver will only become possible with the right approach. This includes not just the traditional steps of development but also success tips and best practices. So, how do you approach an AI PoC development project to ensure the best outcomes? Matt Kurleto, the CEO of Neoteric, knows exactly how:
“In my upcoming book, I present an AI Innovation Funnel that aligns with business strategy while providing a structured approach to experimentation. Here are some key tools, techniques, and best practices:
1. Start with Strategy, not Technology
First, identify the business need standing behind the idea of implementing AI. Clearly define what problem you’re trying to solve and how AI might help. Sometimes, the best decision is not to build an AI model if it doesn’t directly tie to your strategy.
- Distinguish between small “‘i” innovations and Big ”I” Innovation. Focus on incremental, small improvements (like predictive maintenance or chatbot assistants) that deliver quick wins, while keeping an eye on the potential for “Big Innovation” disruption (like completely reimagining how an industry operates).
- Embrace iterative PoC design. Build a minimal viable model — like training our “AI intern” — to test your key assumptions. Gather real-time feedback from end-users and iterate fast. Use clear KPIs (e.g., cost savings, error reduction, time to resolution) to decide if a PoC should move forward or pivot.
2. Take Care of Your Data Pipeline
Clean, consistent data is the lifeblood of AI. Poor data quality is a leading cause of PoC failure — so make sure that you have it under control. Then, as you scale from PoC to production, remember to ensure data privacy and regulatory compliance.
3. Manage Stakeholders’ Alignment, Engagement, and Expectations
Clearly define what “success” looks like — improved customer satisfaction, reduced downtime, or a new revenue stream — and make sure the sponsoring business unit, end-users, and technical teams share these success criteria and a common vision. Early demos, workshops, and open feedback channels will help you maintain their buy-in.
4. Scale the Successful PoCs
If a PoC shows strong ROI or strategic value, prioritize it for further investment. Create a roadmap for broader adoption, training, and infrastructure upgrades. Remember that failures are also part of the process. Learn, document why something failed, and use those insights to refine the next iteration.
And finally, the most important one: Remember that AI is a “force multiplier.” If you apply it to efficient, well-aligned processes and teams, it accelerates success. If you apply it to disorganized or misaligned operations, it can amplify inefficiencies. The AI Innovation Funnel ensures you’re methodical — start small, learn fast, and then scale.”
Real-World Examples of Successful AI PoC Implementation
The decision to develop and implement an AI solution is not always an easy one when you don’t know where the project will be in the end. This is why a Proof of Concept can be such a lifesaver: with a reasonable use of time and money, it allows teams to quickly see their idea in action. Here are three real-life examples of companies we’ve worked with successfully completing their AI PoC journeys.
1. Chatbot with a Document Search Feature
The first project integrates a document search model into a chatbot, allowing for efficient information retrieval. Using Python, microservices, LangChain, and AI models like OpenAI GPT-4 and Anthropic Claude 3, the team deployed the system on Kubernetes for maximum scalability and reliability.
Key features of the solution include a chatbot with document search, conversation, file uploads, and keyword search with source page previews, which enables seamless interaction and precise information retrieval.
The use cases include legal firms searching for documents, HR departments reviewing policies, and financial institutions accessing compliance reports. The solution provides instant document access with reference points, enhancing workflows across various industries.
2. On-Premise Text-to-Speech Solution
The challenge of the next project was to teach the model to replicate the voices of different celebrities. The solution involved creating a high-quality text-to-speech system with customizable voices. Using technologies like text-to-speech and transformers, along with custom AI voice cloning models, the system was integrated with both cloud and on-premise systems.
Key features implemented throughout the project include the ability to select languages, choose celebrity speakers, and input any text to be synthesized. This setup allows for a wide range of customizable voice options for different applications.
The solution is deployed in customer service automation for virtual assistants, media localization for multilingual voice-overs, and e-learning platforms for multilingual content. It enhances customer experience in automated service lines by providing more natural and engaging interactions.
3. Face Swap & Lip Syncing Application
On the final project, the challenge was to achieve realistic face integration while preserving natural expressions in video content. The solution includes precise face replacement and lip-sync features, ensuring seamless visual and audio alignment. Using deep learning and computer vision technologies, along with advanced face-swap models, the system integrates easily into video editing tools.
Key features include using source videos for face replacement, lip-syncing, and audio alignment, as well as swapping faces and audio from target sources. This enables effortless customization of video content with high accuracy.
The potential uses for this solution include film production for visual effects, rapid face-swapping for media companies, social media content editing, and real-time face-swapping in live broadcasts and entertainment events. This technology streamlines video editing while maintaining high-quality results.
Final Thoughts
Sooner or later, adopting AI technology is going to be something most companies will need to at least consider. Artificial intelligence can help organizations offer new services, reach out to a wider audience, achieve higher operational efficiency, and gain a valuable competitive advantage in the increasingly grueling market. AI PoC is the best way to take a product idea for a spin, better understand its potential, and learn valuable lessons to use in a full AI development project. Hopefully, our practical tips and market insights will help you make all the right decisions when it comes to turning your AI idea into a functional product.
- What Is an AI Proof of Concept?
- When to Use AI PoCs and What Can You Gain from AI Proof of Concept?
- Key Use Cases of AI Proof of Concept
- Why Businesses Need AI PoC: The Biggest Benefits AI PoCs Offer
- How to Develop a Proof of Concept in AI: 5 Steps for Building an AI Solution PoC
- Common Challenges of AI Development at the PoC Stage
- How to Maximize the Success of an AI PoC Development
- Real-World Examples of Successful AI PoC Implementation
- Final Thoughts
Planning to improve development process?
Decrease the entropy of product design and development, and increase your chances of success.
Written by
We Help With
Your tech partner needs to be well versed in all kinds of software-related services. As the software development process involves different stages and cycles, the most natural solution is to have them all performed by the same team of experts. That’s exactly what our diverse range of services is for.
The choice of technology for your software project is one of the defining factors of its success. Here at QArea, we have hands-on experience with dozens of popular front-end, back-end, and mobile technologies for creating robust software solutions.
In-depth familiarity and practical experience with key technologies are one of the cornerstones of successful software development and QA. But it also takes specific knowledge of the industry to develop a solution that meets the expectations of the stakeholders and propels its owner to success.

Ensure an effective online presence for your business with a corporate site.