AI in Banking and Finance Sector
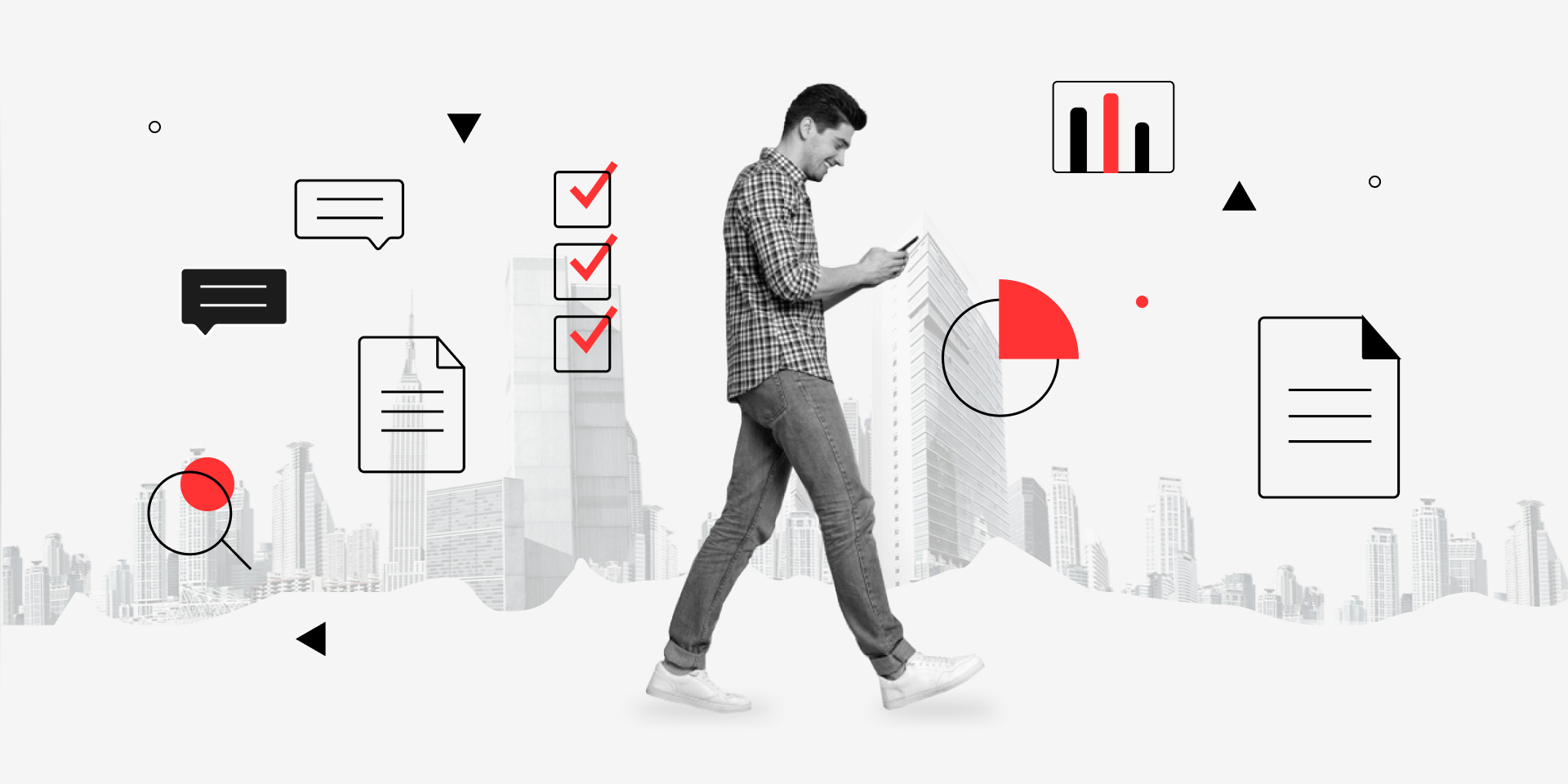
When asked how difficult it is to convince CEO and board members at J.P. Morgan to heavily invest in AI in banking, Derek Waldron, Chief Analytics Officer, says:
“No convincing is necessary. We all know AI is the future of the industry.“
J.P. Morgan is a guiding star in tech advancement for traditional banks. What they do now, most will implement in the upcoming 2–5 years.
Not having J.P. Morgan’s resources doesn’t mean passing on AI advancement. The key is to focus on a deliberate approach, balance risk vs. revenue, and identify areas where AI implementation will have the most impact from the start.
This article will cover use cases, risks, and options for AI in banking. Most importantly, we will get into tech behind AI promises.
But let’s start with the most important question — why does it all matter for the key stakeholders? What do providers, users and regulators get from rapid advances in AI?
For providers
The use of artificial intelligence in banking started not from making customer experience better, but from improving how accuracy and speed of dealing with large volumes of data and credit card fraud and beyond.
Data-informed banking solutions. Digital finance world is full of data, and analyzing that data in real-time comes with customers’ higher satisfaction and extra financial gains. AI helps providers to analyze vast amounts of data faster and more efficiently, find actionable insights, and offer services that are both user-centered and profitable.
New-level customer engagement. By understanding client behaviors, AI allows providers to offer solutions that resonate, building stronger relationships and ensuring customer loyalty.
Risk mitigation. AI models provide a clearer financial foresight, aiding providers to make more informed lending decisions and ensuring compliance.
Operational elevation. AI’s impact is evident across every aspect of banking operations. From automating mundane tasks to enhancing decision-making processes, from enhancing anti-money-laundering measures and anti-terror operations to streamlining back-office activities, AI plays a vital role at every level, according to SAS Insights.
But what is there for users when it comes to benefits of AI in banking? Which products and services get better for us and clients, and indicate that the banking sector relies on the opportunities presented by AI.
For users
It goes beyond AI-powered chatbots and virtual assistants, or even loan and credit operations. Before we get to detailed and very technical AI use cases in banking, let’s see real-world impact clients can feel already or will in the nearest future.
Data-informed banking experience. Everything we do leaves a digital footprint. Every transaction, every click, tells a story. AI helps banks and financial institutions make sense of all this information, turning overwhelming amounts of data into smart decisions.
Personalization. Gone are the days of generic banking services. AI helps to design services tailored to your financial habits and goals, making your banking experience feel, well, more “you.”
Safety and assurance. The financial world has its share of pitfalls. AI serves as a vigilant guardian, predicting challenges and safeguarding against fraudulent activities. It is used in security testing, fraud prediction and assessment of potentially malicious users.
Efficiency at its best. The endless queues at banks and the long hold times on calls? Those are gradually becoming tales of the past, thanks to AI-driven automation ensuring faster and smoother operations.
For Regulators
Regulators play a crucial role in ensuring that the rapid adoption of AI in banking is both safe and compliant.
Enhanced monitoring. AI helps regulators track income and expenses across banks, allowing them to detect and prevent fraudulent activities more effectively.
Streamlined compliance. AI-driven tools can automate the analysis of complex regulations and their application across various use cases in the banking sector.
Improved risk management. By analyzing patterns and anomalies comprehensively, AI supports regulators in safeguarding the financial system from potential threats, ensuring a more stable environment for both providers and users.
Proactive oversight. With AI, regulators can move from a reactive to a proactive stance, anticipating issues before they arise. This shift enables them to guide banks more effectively in adopting AI technologies that enhance customer experiences while maintaining compliance with regulatory requirements.
The benefits are undeniable, but to fully appreciate how AI is transforming banking, we need to look at the specific technologies making it all happen.
And the best place to start, especially with all the hype and noise, is by distinguishing between Traditional AI and Generative AI. Understanding these key differences will help clarify why both are essential in the evolving landscape of banking and finance.
Traditional AI and Generative AI in Banking and Fintech
When people talk about artificial intelligence in banking and beyond today, they often mean Generative AI (Gen AI) because it’s made a lot of noise recently.
However, to understand how AI works in banking, it’s important to distinguish between traditional AI and Generative AI (Gen AI).
Traditional AI in Banking
- Has been used for years
- Focuses on specific tasks like fraud detection, credit scoring, and risk assessment
- Uses structured data (numbers, categories) from bank databases
- Relies on statistical models and machine learning algorithms
- Provides specific outputs based on predefined rules
The mentioned J.P. Morgan already invested more than $2 billion in data management and optimization, part of which was done based on traditional AI — machine learning models, AI-enhanced cloud computing, etc.
Generative AI (Gen AI) in Banking
- Is a newer technology, gaining attention since 2022
- Uses large language models (LLMs) like GPT
- Can process and generate human-like text
- Handles unstructured data like customer queries or documents
- Can be used for chatbots, content creation, and summarizing information
Both types of AI in the banking industry are highly valuable. Traditional AI continues to power many core banking functions, while Gen AI, in real time, opens up new possibilities for customer interaction and data analysis.
Understanding this difference helps in seeing the full picture of AI in banking and finance, both today and in the future.
Traditional AI is already a huge part of modern banking; Generative AI is being implemented and tested and has huge potential but requires more attention to the risks it brings in bias, regulatory compliance, and overall data security.
Transform Your Banking Tech Stack: Partner with QArea’s AI Experts!
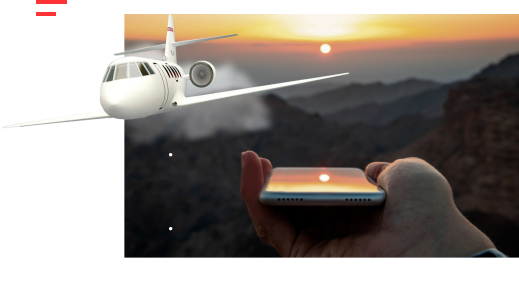
- lex transaction networks
- Unsupervised learning for detecting unusual patterns
- Natural Language Processing (NLP) for analyzing unstructured data in transaction descriptions
Graph analytics can uncover hidden relationships between accounts, detecting complex schemes where money is funneled through multiple small transactions across different accounts to avoid detection.
Algorithmic Trading
- Reinforcement learning for adaptive trading strategies
- High-frequency time series analysis for market microstructure insights
- Sentiment analysis of news and social media for trading signals
16 Traditional AI Use Cases in Banking and Fintech
Here, we both try to please readers on the tech and business side. To show how this “sausage” is done and what you can actually get in the end.
Fraud Detection
- Anomaly detection algorithms and supervised learning models (e.g., Random Forests, Gradient Boosting Machines)
- Transaction patterns, location data, and user behavior in real-time
- Rule-based systems combined with machine learning for adaptive detection
Anomaly identifying algorithms might flag a transaction where a customer’s card is used in two different countries within a short time, prompting the bank to temporarily freeze the account and alert the customer.
Credit Scoring
- Logistic regression, decision trees, and ensemble methods
- Alternative data sources (e.g., social media, utility bills) for a comprehensive assessment
- Machine learning for continuous model improvement based on repayment behaviors
Using alternative data sources, such as social media activity, can help assess creditworthiness for individuals who have limited traditional credit history. For example, consistent payment of utility bills might positively impact the credit score of someone with a thin credit file.
Risk Management
- Monte Carlo simulations and Value at Risk (VaR) models
- Time series analysis for market risk prediction
- Bayesian networks for operational risk assessment
Monte Carlo simulations could be used by a bank to stress test its portfolio under various economic scenarios, helping to identify potential vulnerabilities if market conditions were to change dramatically.
Anti-Money Laundering (AML)
- Graph analytics to identify comp
Sentiment analysis might analyze millions of tweets and news articles to gauge public sentiment towards a particular company, influencing the trading algorithms to buy or sell the stock before market sentiment shifts.
Customer Segmentation
- Clustering algorithms (e.g., K-means, DBSCAN) on multi-dimensional customer data
- Dimensionality reduction techniques like PCA for feature selection
- Hierarchical clustering for nested segment identification
Clustering algorithms could group customers based on spending habits, allowing the bank to tailor marketing campaigns. For instance, a cluster of high-spending customers on travel might receive offers for premium travel rewards credit cards.
Predictive Analytics
- ARIMA and SARIMA models for time series forecasting
- Deep learning (LSTM networks) for complex pattern recognition in financial data
- Ensemble methods for robust predictions across various financial indicators
Deep learning models might predict stock price movements based on historical trading data, allowing the bank to make informed decisions on buying or selling assets.
Blockchain and Distributed Ledger Technology (DLT)
- DLT provides a secure, decentralized way to record and verify transactions
- Smart Contracts automate the execution of contract terms when predefined conditions are met
- Consensus Algorithms like Proof of Work (PoW) or Proof of Stake (PoS) ensure agreement across distributed networks, maintaining the integrity of the ledger
Cross-border payments and settlements. By using blockchain, banks can significantly reduce the time and cost associated with cross-border transactions. For example, projects like J.P. Morgan’s Quorum allow for near-instantaneous settlements, reducing reliance on traditional intermediaries and enhancing transparency.
Robotic Process Automation (RPA)
- Optical character recognition (OCR) for document processing
- Rule-based systems for workflow automation
- Machine learning for adaptive process optimization
OCR in document processing could automatically extract information from loan applications, speeding up the approval process and reducing the need for manual data entry.
Personalized Financial Advice
- Collaborative filtering and content-based recommendation systems
- Reinforcement learning for dynamic portfolio optimization
- Multi-armed bandit algorithms for balancing exploration and exploitation in recommendations
Collaborative filtering could suggest investment options to customers based on the behavior of other similar customers, offering a more personalized portfolio that aligns with their risk tolerance and financial goals.
Chatbots for Basic Queries
- NLP techniques like named entity recognition and intent classification
- Retrieval-based and rule-based systems for predefined responses
- Dialogue management systems for maintaining context in conversations
NLP techniques enable chatbots to handle common queries like “What’s my account balance?” or “How do I reset my password?” with immediate, accurate responses, improving customer service efficiency.
Anomaly Verification
- Isolation forests and one-class SVM for identifying outliers
- Autoencoders for unsupervised anomaly detection in high-dimensional data
- Time series decomposition techniques for detecting seasonal anomalies
Autoencoders might detect an unusual spike in transaction frequency from a typically low-activity account, triggering further investigation to prevent potential fraud.
Credit Card Approvals
- Ensemble methods (Random Forests, XGBoost) for binary classification
- Feature engineering to create complex indicators from raw financial data
- Model interpretability techniques (e.g., SHAP values) for regulatory compliance
Model interpretability techniques ensure that when a credit card application is denied, the bank can clearly explain the decision to the applicant, enhancing transparency and customer trust.
Loan Underwriting
- Survival analysis models for estimating loan default probabilities
- Gradient boosting machines for risk assessment
- Cost-sensitive learning to balance risk and approval rates
Cost-sensitive learning might prioritize loan approvals for small businesses during a downturn, balancing the risk of default with the potential economic benefits of keeping businesses afloat.
Compliance Monitoring
- Text classification algorithms for regulatory document analysis
- Graph analytics for detecting complex compliance violations
- Anomaly detection techniques for identifying unusual reporting patterns
Text classification algorithms could automatically flag regulatory documents that contain potentially non-compliant language, allowing compliance officers to review and address issues proactively.
Customer Churn Prediction
- Survival analysis and Cox proportional hazards models
- Recurrent neural networks for sequence prediction of customer behaviors
- Feature importance analysis for identifying key churn factors
Recurrent neural networks might predict when a high-value customer is likely to leave based on their recent interactions, allowing the bank to offer incentives to retain them.
These AI use cases are not exhaustive, though. Here are some more real-world examples of AI applications in banking big players rely on:
- Bank of America uses machine learning to detect fraud flagging unusual transactions and alerting customers to potential breaches.
- J.P. Morgan relies on NLP to analyze legal documents in seconds, a process that traditionally took human experts thousands of billed hours.
- Santander was one of the first banks to introduce a blockchain-based money transfer service, allowing customers to make international transfers within a day.
Experience Matters: Explore QArea’s Case Studies in AI-Driven Banking and Fintech!
15 Banking and Fintech Generative AI Use Cases
Advanced Chatbots
- Large language models (LLMs) like GPT for natural language understanding and generation
- Contextual embeddings for maintaining conversation history
- Few-shot learning for handling domain-specific queries
Large language models (LLMs) like GPT could allow a bank’s chatbot to not only answer customer queries but also engage in complex conversations about investment strategies or mortgage options, simulating a more personalized and in-depth consultation.
Content Creation
- Transformer-based models for generating human-like text
- Text-to-text transfer learning for financial domain adaptation
- Abstractive summarization techniques for concise report generation
Transformer-based models might generate personalized financial newsletters for customers, summarizing their recent transactions, market news, and providing tailored investment advice based on their portfolio.
Personalized Financial Planning
- Multi-task learning models to handle various financial planning aspects
- Reinforcement learning for optimizing long-term financial strategies
- Attention mechanisms for focusing on crucial financial factors
Reinforcement learning could help create a financial plan that adjusts in real-time to market changes and life events, offering advice on when to save more aggressively or when to invest in higher-risk opportunities.
Document Analysis
- BERT-based models for document understanding and information extraction
- Graph neural networks for capturing document structure
- Extractive and abstractive summarization techniques
BERT-based models could automatically review a large batch of financial documents, such as loan agreements, to extract and highlight key clauses or potential risks, saving hours of manual work for compliance officers.
Contract Review
- Named entity recognition for identifying key contract elements
- Zero-shot learning for handling various contract types
- Semantic similarity measures for clause comparison and anomaly detection
Zero-shot learning might be used to quickly review and compare contracts from different vendors, identifying any unusual clauses or discrepancies that might otherwise be overlooked by a human reviewer.
Fraud Prevention
- Generative adversarial networks (GANs) for simulating new fraud scenarios
- Graph convolutional networks for analyzing transaction patterns
- Anomaly detection in the latent space of autoencoders
Generative adversarial networks (GANs) could simulate new and sophisticated fraud scenarios that haven’t been seen before, allowing banks to develop defenses against these potential threats before they occur.
Market Sentiment Analysis
- BERT-based models fine-tuned on financial texts for sentiment classification
- Aspect-based sentiment analysis for detailed market insights
- Transferring learning from pre-trained finance-specific language models
Aspect-based sentiment analysis could provide a detailed breakdown of how different sectors or companies are perceived in the market, enabling traders to make more informed decisions about their investments.
Automated Reporting
- Seq2seq models with attention for data-to-text generation
- Controllable text generation for customizing report styles
- Multi-modal models for incorporating charts and tables in reports
Multi-modal models might generate comprehensive financial reports that include both text summaries and visual elements like charts and graphs, offering a more holistic view of a client’s financial status.
Product Recommendation
- Transformer-based models for understanding customer queries and preferences
- Collaborative filtering in the embedding space of customer-product interactions
- Multi-armed bandits for balancing exploration and exploitation in recommendations
Transformer-based models could analyze a customer’s spending habits and preferences to recommend new financial products, such as a credit card with travel rewards for a frequent traveler, enhancing customer satisfaction.
Customer Support Improvement
- Topic modeling (e.g., LDA, BTM) for categorizing customer issues
- Sentiment analysis on customer interactions for emotion tracking
- Sequence-to-sequence models for generating improved response templates
Sentiment analysis on customer interactions could detect when a customer is becoming frustrated during a support call, triggering the system to escalate the issue to a human agent or offer a more empathetic response.
Risk Assessment Narratives
- Controlled text generation for tailoring explanations to different audience levels
- Knowledge graph embeddings for linking risk factors in explanations
- Style transfer techniques for adapting technical content to layman terms
Style transfer techniques might allow risk assessment reports to be automatically adapted to different audiences, such as simplifying technical details for a non-expert client or adding more data-driven insights for an analyst.
Financial Education
- Adaptive learning algorithms for personalizing educational content
- Reinforcement learning for optimizing learning paths
- Text simplification models for adapting complex financial concepts
Text simplification models could be used to create easy-to-understand explanations of complex financial concepts for customers, helping them make more informed decisions without needing a finance degree.
Scenario Analysis
- Variational autoencoders for generating diverse financial scenarios
- Monte Carlo tree search for exploring decision outcomes
- Transformer models for narrative generation of scenario descriptions
Variational autoencoders could generate a wide range of possible economic scenarios, helping financial planners and clients understand how different strategies might perform under various conditions, such as a market downturn.
Code Generation
- Transformer-based models trained on code repositories (e.g., CodeX)
- Abstract syntax tree (AST) parsing for code understanding and generation
- Reinforcement learning for optimizing generated code quality
Transformer-based models trained on code repositories might allow banks to automatically generate scripts for routine data processing tasks, reducing the need for manual coding and speeding up deployment times.
Voice Banking Assistance
- End-to-end speech recognition models (e.g., wav2vec, HuBERT)
- Text-to-speech models (e.g., Tacotron, WaveNet) for natural responses
- Dialogue state tracking for maintaining context in voice interactions
End-to-end speech recognition models could enable a voice assistant to handle complex banking tasks, like setting up a new savings account or transferring funds between accounts, all through natural conversation, making banking more accessible for those who prefer voice interaction.
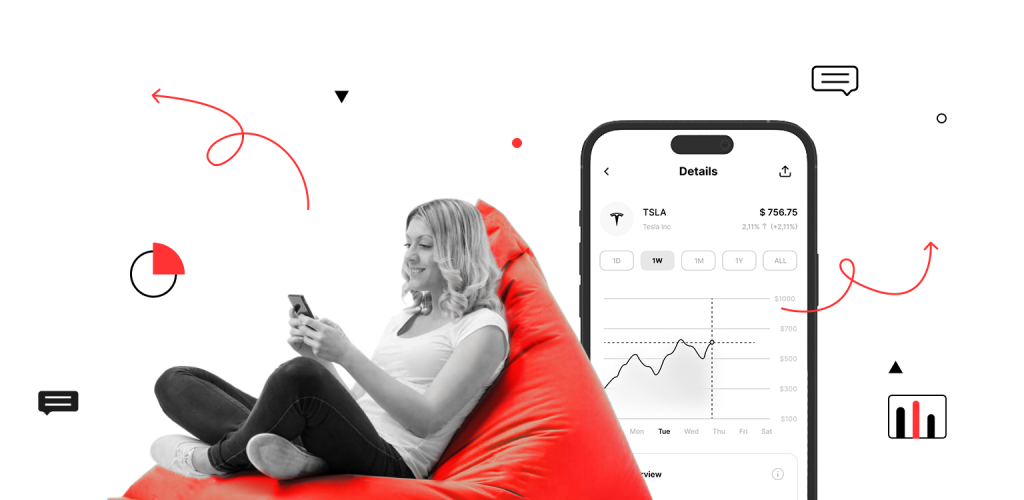
Applications of AI in Banking: Real-World Examples
We have just covered the core technologies powering the AI revolution in banking and finance sector. You might wonder why we’d circle back to the same territory. The reason is perspective.
While understanding the tech behind the scenes is crucial, there is a chance you are reading this article not with a distinct business purpose. You may be looking to develop a cutting-edge financial solution, deploy a new banking product, or enhance your existing system. So what you really want to know is how to align adoption of AI capabilities with specific banking products.
Know Your Customer (KYC)
Truly knowing your customer is both a regulatory mandate and a smart business move. AI makes the task less of a chore and more of a charm.
- Automated document verification. KYC typically involves verifying heaps of documents. Adopting AI can help swiftly scan, verify, and categorize these documents, ensuring that customer onboarding is both fast and compliant.
- Behavioral analysis for red flags. It’s not just about initial verification. AI can also monitor transactions to spot unusual behaviors or patterns. If Mr. Smith suddenly sends a huge sum overseas, AI suspects a “stolen credit card” case and raises the flag for further review.
- Facial recognition for live verification. With AI, a live video or selfie can cross-check faces with stored ID photos, offering an additional layer of verification that’s both user-friendly and secure.
- Continuous data update. People’s lives change – they move, get married, change jobs. AI can prompt customers to update their details, ensuring that the bank’s data is as current as possible. For providers, this means always staying compliant without the manual hustle.
We will circle back to some of these functions in the following operational and product aspects. Bear with us, as fintech is an interconnected web, where you can’t just fully separate KYC and fraud prevention functions and solutions.
Checking and Savings Accounts
Checking and savings accounts, not just a place to keep money. AI helps to turn these classical banking products into smart financial tools.
- Personal financial management. AI can analyze spending habits, categorize transactions, and offer budgeting insights, giving your banking mobile app a leg up on your competitors.
- Anomaly detection. Beyond the standard unusual activity alert, think of a system that learns a user’s spending habits over time. Red flags now can consist of making purchases in unusual hours, spending week-worth budget in an irrelevant, never visited before online-store, repetitive side service request for a verification transaction, services not consistent with the client’s spending style.
- Future spend predictions. Financial guru and wannabes advise people to budget as the first step to financial stability and freedom. In reality, it is close to advising “eating healthier” — generic and hard to follow consistently. A predictive model in your app could forecast a user’s balance at the end of the month based on their recurring bills and typical spending and help with better financial planning.
Credit Cards
- Context-aware spending analysis. AI can detect the context and the anomalies, analyzing card transactions in relation to real-world events. For example, if there’s a spike in spending while you’re on vacation, the system understands the context, reducing false alerts for unusual spending while still monitoring for genuine fraud.
- Automated savings round-ups. By integrating AI, cards can help users save. Each transaction can be rounded up to the nearest dollar (or $10), with the difference automatically transferred to a savings account. Over time, AI can even suggest adjustable saving rates based on spending patterns and saving goals for a defined period.
- Adaptive card locking. AI can predict when you’re less likely to use your card — like during your regular sleeping hours — and auto-lock it to prevent any unauthorized access. In case of genuine transactions during these hours, it learns and adjusts.
Loans and Mortgages
With AI, financial institutions can provide a smoother, more informed borrowing experience, increasing chances to get loans even without great credit history and protecting lenders from malicious users.
- Seeing the full picture in credit scoring. AI helps banks look at more than a clients’ loan or credit history to determine their creditworthiness. It can consider things like your shopping habits, online activity, and more to get a better idea of how good you are with money.
- Quick loan approvals. No one likes waiting. With AI, loan approvals can be almost instant, making customers happy about this particular financial institution and allowing banks to do more business thanks to the word of mouth marketing both online and offline.
- Spotting loan issues in advance. AI can help banks guess when someone might struggle to repay a loan, way before the person themselves realize it. This way, they can check in early and figure out a plan before things get messy.
- Fair play in lending. Sometimes, without even meaning to, people and systems have biases. This can lead to unfair loan decisions. AI can be trained to minimize these biases, ensuring everyone gets a fair shot.
Investment Services
Using AI for investment services in a banking product helps to ensure both fresh-faced and veteran investors stay on course.
- Tailored robo-advisors. AI-driven robo-advisors aren’t just smart; they’re intuitive. They learn about client’s financial goals and habits. This means customers get personalized advice, boosting trust and long-term loyalty to your bank or firm.
- Market pulse with Sentiment Analysis. AI solutions fish out valuable insights from news, social media, and financial forums. Offering this intel to your clients can give them an edge, positioning your service as a go-to source for market foresight.
- Automated portfolio management. Manual portfolio rebalancing feels so last decade. With AI, portfolios auto-adjust based on market trends. For providers, this means fewer human errors, increased efficiency, and a modern service that attracts tech-savvy investors.
Digital Wallets and Payment Systems
You and your clients constantly have money on the move. AI ensures this dance of digits is swift, secure, and smart.
- User payment predictions. AI observes and learns user habits. For banks and fintechs, this means anticipating client needs, and rolling out tailored financial products or timely offers.
- Route optimization for transactions. AI finds the quickest, most efficient path for transactions, cutting down wait times and increasing customer satisfaction.
- Smart promotions. Based on user spending and habits, AI applications can suggest the right deals and discounts at the right time, increasing transaction volumes.
Trade Finance
If you want to roll out a separate trading solution or incorporate it in into the existing banking product, don’t overlook possible AI integrations.
- Automated document verification. Checking and cross-referencing trade documents is old school. AI speeds up the process, reducing errors and ensuring smoother trade operations.
- Trade risk forecasts. With AI’s analytical prowess, foreseeing potential trade hiccups becomes significantly easier. For providers, this means better risk management, ensuring your clients’ trust remains intact.
- Currency conversion. AI algorithms keep an eagle eye on currency fluctuations, ensuring optimal conversion rates. It’s about maximizing value with every transaction, making your financial platform the preferred choice for businesses.
Asset Management
- Algorithmic valuation. AI evaluates assets faster and makes accurate and current valuations based on a multitude of factors.
- Market movement forecasting. Instead of gut feelings or broad market trends, AI analyzes vast data to forecast precise market shifts.
- Automated asset allocation. Tailoring investment portfolios becomes hassle-free as AI auto-allocates assets based on specific strategies and goals.
Foreign Exchange and Remittances
- FX rate prediction. AI digs into past trends, current news, and more to predict potential FX rate movements.
- Remittance timing. By understanding the FX market’s ebb and flow, AI can suggest the most cost-effective times for international money transfers.
- Automated conversion. AI swiftly processes conversions, and users get the most bang for their buck, linking this speed and efficiency to the quality of your product.
AI in Banking and Fintech: Practical Scenarios and Tools
AI can transform various aspects of financial services, whether you’re a startup or an established bank. Here’s how different organizations might apply AI tools and technologies to achieve their goals:
Niche Fintech Startup: AI-Powered Budgeting Tool
Your fintech startup is developing a budgeting tool tailored to freelancers. To stand out, you might use Natural Language Processing (NLP) for chatbots that answer user questions and offer financial advice. Reinforcement learning could help the app adapt to users’ spending habits over time, providing more personalized recommendations. Predictive analytics might forecast income variability for freelancers, helping them plan better.
Regional Bank: Digital Services with AI-Driven Support
As a regional bank entering the digital space, you’re launching a mobile app with features like automated customer support and spending analysis. For this, you could implement NLP and dialogue management systems to create chatbots that handle basic customer queries efficiently. Anomaly detection algorithms could be used to monitor transactions for unusual patterns, enhancing security. Collaborative filtering might help the app offer personalized financial tips based on user behavior.
Large Bank: Expanding into New Markets
Expanding into international markets requires launching AI-driven tools for loans, credit scoring, and customer service. Logistic regression and decision trees could be used for quick and accurate credit scoring. Sentiment analysis might analyze local news and social media to gauge market sentiment and adapt your offerings accordingly. For customer service, LLMs (Large Language Models) like GPT could help create chatbots that handle diverse customer queries in multiple languages, ensuring smooth interaction in different regions.
Mid-Tier Bank: Enhancing Security with AI
Security is a top priority as you implement advanced AI-based fraud detection systems. Anomaly detection algorithms could be employed to spot suspicious transactions in real-time. Graph neural networks might be used to analyze complex transaction patterns and uncover potential fraud schemes. To simulate new fraud scenarios and prepare defenses, Generative Adversarial Networks (GANs) could be valuable.
Credit Union: Upgrading Member Services with AI
Your credit union aims to introduce AI-driven financial planning tools for better member service. Multi-task learning models can handle various financial planning aspects, offering personalized advice based on each member’s financial history and goals. Attention mechanisms could be used to focus on key financial factors, helping members make informed decisions. Text simplification models might adapt complex financial information into easy-to-understand content for your members.
Digital-First Fintech: Scaling AI Capabilities
As a growing fintech, scaling your AI capabilities is crucial. You might use deep learning models to enhance customer support with more accurate responses and recommendations. Clustering algorithms could help segment your user base, allowing for more targeted marketing and product offerings. For security, autoencoders might detect anomalies in transaction data, ensuring safety as you expand.
Matt Kurleto on AI in Banking and Finance
While AI implementation in banking and fintech holds immense potential, the reality of executing these strategies can be complex.
It’s easy to get caught up in the allure of cutting-edge technology, but as with any major initiative, the devil is in the details. Implementing AI solutions that look great on paper can sometimes lead to unexpected challenges and even hinder a company’s growth if not aligned with the core business goals.
To shed light on the nuances of successful AI integration, we spoke with Matt Kurleto, CEO & Founder of Neoteric and a seasoned AI Strategy Workshop Facilitator. Matt emphasizes the importance of aligning AI initiatives with clear business objectives to ensure they drive real value.
“One of the biggest mistakes I see is implementing wrong solutions. When the company is focusing on growth, implementing an AI solution that cuts costs distracts you from the main goal and actually slows you down.
AI implementations should be aligned with company goals and clearly deliver monetary value to the company. Increase Customer LTV, Cut time to close the deal, and increase conversions from the cart.
To implement it successfully, you need to start with what you can improve in your business rather than going through a list of random AI content generators for your emails, tasks, customer support, blog, and whatever.
If you have more ideas to support your organization with AI governing, it is the key point to building AI Strategy that consistently enables the company to innovate with AI.”
Matt Kurleto, CEO & Founder of Neoteric, AI Strategy Workshop Facilitator
and link: https://aineoteric.com/
Adopting AI in the Banking Sector: Future Prospects
The future of AI in banking lies at the intersection of advanced technology and consumer needs. As AI becomes more intertwined with finance, several groundbreaking trends are emerging.
Modernizing legacy systems. For banks to even consider using AI technology efficiently, they need to upgrade their core systems. A hybrid, multi-cloud strategy allows combining the best of AI’s scalability with the security of private clouds and on-site setups.
Rise of super apps. Traditional banking apps focused on specific functions. The future lies in super apps, single platforms integrating banking, shopping, payments, investments, and more. This consolidation offers customers convenience and streamlines operations for providers.
Embedded finance. No longer a stand-alone sector, financial services will be deeply integrated into non-financial platforms. Think of checking out on an e-commerce site and getting instant loan offers for your purchase — all powered by AI.
AI-driven financial health checkup. Just as you have a health checkup, AI will offer financial checkups, analyzing your financial behaviors, predicting future challenges, and offering advice to keep you on track.
Blockchain and AI convergence. The secure nature of blockchain combined with the intelligence of AI can reshape financial operations, especially in fraud detection and international transactions.
Augmented Reality (AR) banking. AR could change the face of banking, literally. Envision checking your account balance or visualizing your spending patterns through AR glasses.
Open banking with AI. As more regions adopt open banking regulations, AI will play a crucial role in analyzing vast data from different financial institutions, offering users consolidated insights and tailored advice.
Sustainability and AI. AI applications in banking can also drive sustainable finance by analyzing environmental, social, and governance (ESG) data, helping banks make more responsible investment decisions.
Ethical and transparent AI. With increasing scrutiny on AI’s decision-making processes, there’ll be a move towards more transparent algorithms, ensuring decisions are fair and free from biases.
AI-enhanced cybersecurity. As the financial sector becomes more digital, AI for banking and finance will play a critical role in strengthening cybersecurity measures in many banks, protecting against sophisticated threats and ensuring the integrity of banking systems.
AI and quantum computing. The convergence of AI and quantum computing holds the potential to revolutionize financial modeling and risk analysis, offering unprecedented speed and accuracy in processing complex financial data.
Wealth management. The use of AI in banking will extend to wealth management, where AI-driven robo-advisors will offer more sophisticated, data-informed investment strategies, making wealth management more accessible and efficient.
By prioritizing the alignment of your technology roadmap with a comprehensive AI strategy and collaborating with software development vendors, banks may position themselves for success in the dynamic landscape of digital financial services.
Smarter Banking, Brighter Future: QArea’s AI Innovations at the Heart of Finance!
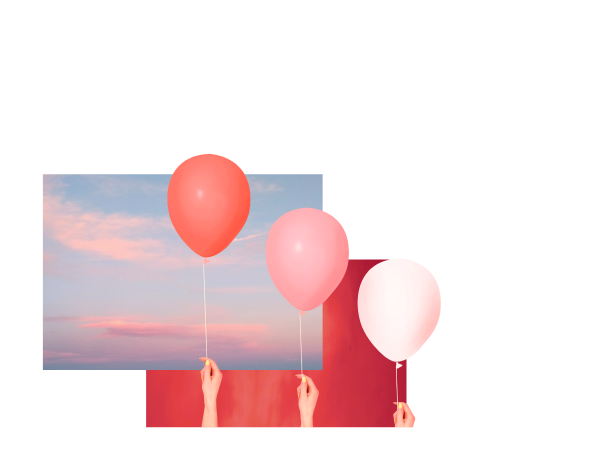
Deploy Your AI-First Bank App: In-House vs Outsourcing
Deciding between in-house or outsourced development for AI-powered banking apps should not be a roll of the dice. Here are some criteria you should consider when planning to use artificial intelligence in banking and finance products.
Criteria | In-House Development | Outsourced Development |
Initial investment | Higher, multifactor | Lower, straightforward |
Infrastructure, software, hiring, onboarding, training, retaining | No need for infrastructure setup; pay for services rendered | |
Domain expertise | Initially limited | Given, proven by various cases |
Requires a separate hire through the full recruitment process, unless AI is a core company’s competency | Dedicated teams with necessary expertise can start in two weeks after the initial request | |
Flexibility and scalability | Limited by the structure | High and controlled by you |
Depends on size & skill set of in-house team, but often highly limited in rigid banking systems | You can swiftly scale team up/down and request some of the experts changed no questions asked | |
Costs | Recurring | Project-based |
Training, salaries, benefits, software licenses | Paying only for specific services and specialists | |
Access to talent | Limited by geography | Wide (near-, on-, offshore) |
Bound to your geographical location and often overprices due to tax and social benefits reasons | Access to a diverse range of experts in AI and supporting dev/QA technologies and languages | |
Time to market | Often longer | Typically faster |
Due to learning curves and potential resource constraints | Expert teams with established workflows and domain experience |
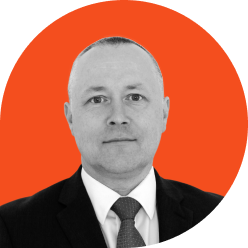
“If you have a legacy-based system in the US or UK to maintain, you are probably paying a stupid amount of money to do it. Train people in other countries, or hire an offshore/nearshore team at least 80% versed in what you do – the support costs will go down. Make sure such decisions are compliant with your SLA. When a company is starting up, they have limited resources and don’t have enough expertise. They may not have domain- or software-based backgrounds, so they need support and guidance. Reduced costs and additional expertise synergize when it comes to outsourcing your project to domain experts.”
10 Key Takeways on Adopting AI in Banking Sector
#1. AI as a must-have. The use of AI in banking is seen as essential for staying competitive, with leaders like J.P. Morgan driving the industry forward.
#2. Strategic adoption. Even without massive resources, companies can implement AI for banking and finance strategically by focusing on high-impact areas like risk management and customer experience.
#3. Enhancing data analysis. AI applications in banking allow for real-time data analysis, leading to smarter, data-driven decisions and more profitable banking solutions.
#4. Improving customer engagement. AI banking solutions enable personalized interactions by understanding client behavior, thereby fostering loyalty and improving user satisfaction.
#5, Risk management. Artificial intelligence in banking enhances risk mitigation through predictive models that help in more informed lending decisions and compliance assurance.
#6. Operational efficiency. AI in banking use cases, such as automating routine tasks and improving anti-money laundering measures, boost operational efficiency and security.
#7. User experience. Use cases of AI go beyond chatbots, offering personalized services, increased safety, and faster, smoother operations, enhancing overall customer satisfaction.
#8. Traditional vs. Generative AI. While traditional AI powers core functions like fraud detection, generative AI is transforming customer interactions and expanding AI applications in banking.
#9. Wide range of AI use cases. AI in banking use cases includes fraud detection, credit scoring, personalized financial advice, and anomaly detection, showcasing its versatility and impact.
#10. Future trends:.The future of artificial intelligence in banking includes modernizing legacy systems, developing super apps, and embedding finance into non-financial platforms, making AI indispensable in the industry.
Transform Your Banking Operations: Explore Outsourcing AI Solutions with QArea!
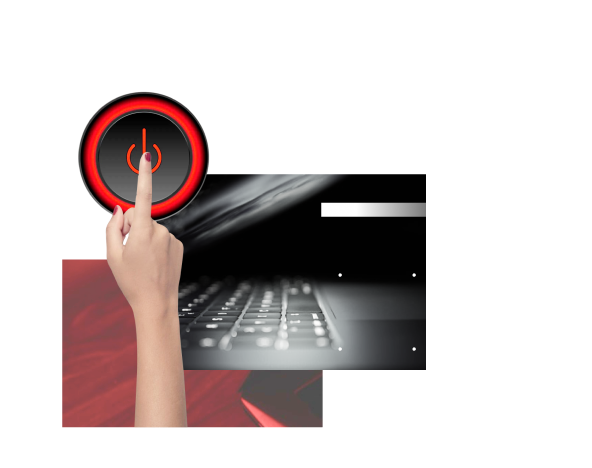
Wrapping Up
ATMs faced initial resistance. People worried about job losses for bank tellers and the safety of their transactions. Wire transfers and neobanks encountered similar hurdles. Yet, these innovations prevailed and became integral to modern banking.
AI in banking is following a similar path. It’s natural for banks, users, and regulators to have concerns. But as with previous innovations, acceptance and integration are inevitable.
We’ve separated traditional AI from generative AI, because this distinction is crucial as there’s often confusion between these two areas.
The banking sector needs to embrace AI. Users will adapt as they see the benefits. Regulators must develop frameworks that ensure safety while allowing innovation.
AI will play a pivotal role in deciding the future of banking and finance. The key is to approach this transformation with an open mind, a focus on security, and a commitment to enhancing the banking experience for all stakeholders.
- For providers
- For users
- For Regulators
- Traditional AI and Generative AI in Banking and Fintech
- 15 Banking and Fintech Generative AI Use Cases
- Applications of AI in Banking: Real-World Examples
- AI in Banking and Fintech: Practical Scenarios and Tools
- Matt Kurleto on AI in Banking and Finance
- Adopting AI in the Banking Sector: Future Prospects
- For providers
- For users
- For Regulators
- Adopting AI in the Banking Sector: Future Prospects
- Deploy Your AI-First Bank App: In-House vs Outsourcing
- Wrapping Up
Planning to improve development process?
Decrease the entropy of product design and development, and increase your chances of success.
Loan Underwriting
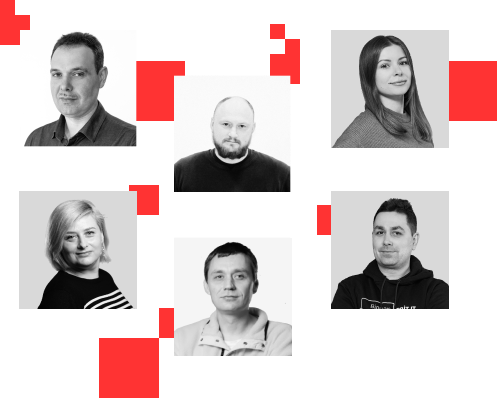
Hire a team
Let us assemble a dream team of specialists just for you. Our model allows you to maximize the efficiency of your team.
Written by
We Help With
Your tech partner needs to be well versed in all kinds of software-related services. As the software development process involves different stages and cycles, the most natural solution is to have them all performed by the same team of experts. That’s exactly what our diverse range of services is for.
The choice of technology for your software project is one of the defining factors of its success. Here at QArea, we have hands-on experience with dozens of popular front-end, back-end, and mobile technologies for creating robust software solutions.
In-depth familiarity and practical experience with key technologies are one of the cornerstones of successful software development and QA. But it also takes specific knowledge of the industry to develop a solution that meets the expectations of the stakeholders and propels its owner to success.

Ensure an effective online presence for your business with a corporate site.