AI in Retail: How to Use Machine Learning in eCommerce
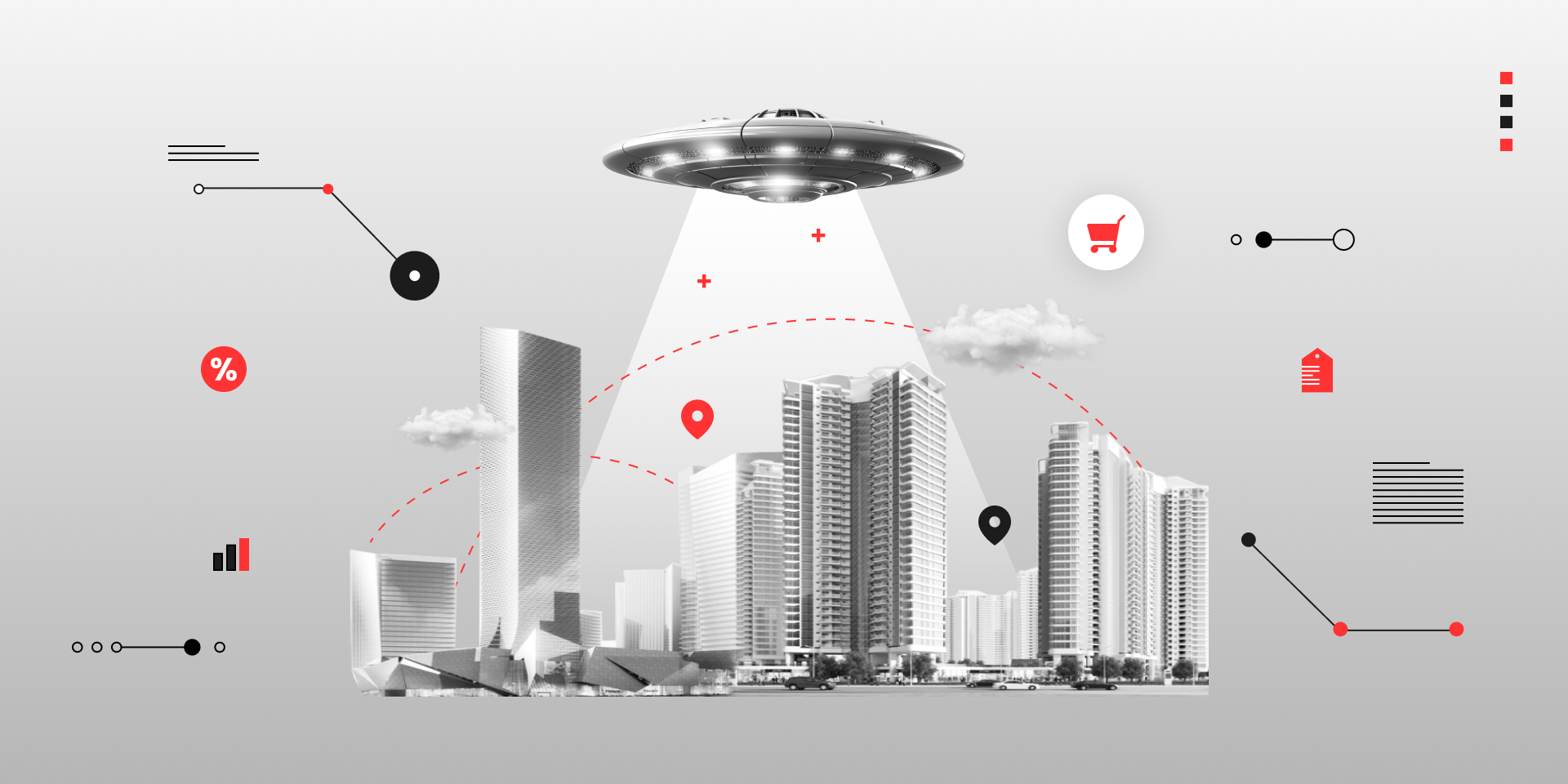
Retail as we know is going to be obliterated by AI. Even Amazon, headless retail’s 800-pound gorilla, can’t rest on its laurels. Supply chains will crumble. Staff will be laid off. Margins will evaporate… or spike! The retail apocalypse is upon us.
But after the carnage, a new era will emerge with intelligence and retail AI solutions at its core. Personalization, discovery, and automation will rule. Survival will depend on who adapts. Right now, only 15% of retailers are using AI, while quantum computing and neural networks advance exponentially. The retail AI market itself is booming, on track to hit $20.05 billion by 2026. This growth, driven largely by personalization and smart product recommendations, is evident with a 230% increase in machine learning investment from 2019 to 2023.
So what does this mean for retail CTOs and technology leaders? Everything.
This article will address what’s possible with AI in eCommerce today — from lightning-fast retail search to psychic-level machine learning recommendations boosting customer experience. Real examples show where the bar is set.
Let’s move to the benefits of machine learning for eCommerce — it seems like there are no problems that cannot be solved by ML algorithms.
Benefits of AI and Machine Learning in eCommerce Business
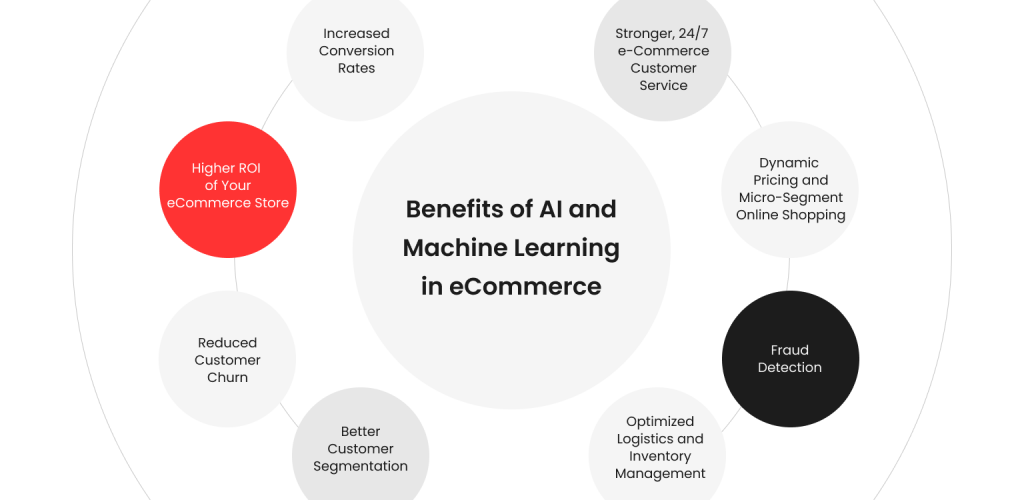
We owe you a disclaimer: you won’t get all the benefits listed below at once. Moreover, before getting any, you will have to invest, make some mistakes and even curse AI revolution a bit.
Higher ROI of Your eCommerce Store
Retailers who have adopted personalization strategies through AI report sales gains between 6-10%, growing at a rate two to three times faster than others. AI’s ability to analyze and adapt to customer habits, preferences, and patterns via AI product recommendations and other tools is central to this increase in sales and customer engagement.
Improving ROI with AI in ecommerce isn’t just about increasing sales, it’s also about cutting costs. By 2030, AI is set to reduce operational expenses by 30%, and by 2035, it’s expected to boost profits by nearly 60%. This points to a major change in how ecommerce businesses will profit by adopting AI.
However, to truly benefit from AI, companies need a solid foundation. High ROI comes from well-implemented AI strategies, including effective data management and ethical practices. Experience in using AI matters too – seasoned companies are seeing a 4.3% ROI, while newcomers only see 0.2%. This highlights the importance of strategic AI use in ecommerce.
Increased Conversion Rates
AI in e-commerce helps to create shopping experiences that turn more browsers into buyers. Discovery AI recommendations and smarter marketing strategies based on machine learning personalization bring a real jump in sales. In fact, AI can boost revenues by up to 15%. It’s about hitting the sweet spot of what customers want, making them more likely to buy. And the numbers back this up — up to 30% of ecommerce revenue now comes from the power of AI and the use of machine learning.
Reduced Customer Churn
AI excels in personalizing customer experiences by analyzing various data points like purchase history and browsing behavior, eCommerce companies feed it. This level of customization not only makes customers feel valued, but also significantly boosts retention. For example, AI can predict customer churn by analyzing subtle changes in customer interactions and purchase frequencies. You get alarmed and can proactively engage at-risk segments with targeted retention strategies. Here some more tools based on ML algorythms that will help you keep customers loyal and happy:
1. Behavioral analysis. Looking at micro-changes in engagement metrics on both aggregate and individual levels:
• Purchase frequency trends over time
• Average order value decreases
• Higher product returns rates
• Fewer page visits or email opens
2. Predictive modeling. Using techniques like logistic regression, random forest classifiers or neural networks to predict the probability of each customer churning based on the above metrics combined with attributes like:
• Purchase history
• Initial source of acquisition
• Demographics and location data
• Length of time as a customer
3. Personalized incentives. Proactively reaching out to high-churn-risk customers with customized promotions and perks matched to their interests and behaviors:
• Targeted product recommendations
• Exclusive sales and bundle pricing
• Free shipping or upgrades
• Birthday surprises and delighters
You know, it just works.
Better Customer Segmentation
Divide customers like an accountant to sell like an artist.
Retailers have always grouped customers into segments like budget shoppers, luxury buyers, suburban moms, and so on. This lets you market effectively based on broader attributes. But in ecommerce, AI lets you subdivide and cluster customers into micro-segments for truly personalized experiences.
Powerful algorithms analyze exponentially more signals from past purchases, browsing patterns, seasonal demand shifts and even sentiment from customer inquiries. They uncover nuanced insights like:
- Budget home chefs who buy premium knives and pans but cheaper pantry staples
- Rural fathers who purchase toys aligned to developmental science
- Upwardly mobile young adults investing in premium athleisure wares
Granular profiling at such individual levels lets you tailor pricing, product recommendations, promotions and more to align with each customer’s interests and sensibilities.
So while old-school segmentation grouped people based on simplistic demographics, AI empowers companies to (here it comes!) divide customers like an accountant to sell to them like an artist. Customers not just enjoy but already expect online shopping customized just for them and you can’t achieve it without unique merchandising strategies personalized down to the SKU.
Get the AI Solution for eCommerce you need to reach your business goals in 2024
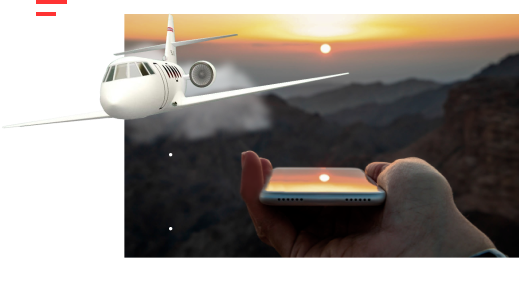
Stronger, 24/7 e-Commerce Customer Service
AI-powered chatbots and virtual assistants fix frustrating customer service with conversational capabilities that:
- Provide instant, 24/7 support on demand rather than limited store hours or call centers;
- Understand questions then respond accurately using natural language processing;
- Access databases to pull up purchase histories and order details when needed;
- Recommend relevant products and surface helpful information;
- Route complex issues seamlessly to human agents.
Take North Face’s customer service bot handles 60% of all inquiries with a “surprisingly high” accuracy (the actual % is still a secret though). Powered by IBM Watson, it fields common asks like order tracking, product questions, returns and more. For trickier conversations, it automatically hands-off the chat to live agents who have full context from the prior bot-customer dialog.
The result is rising satisfaction — 62% prefer chatbots for quick convenience without waits. And they yield strategic insights – machine learning detects rising trends and sentiment shifts from analysis across bot conversations.
So AI delivers the model of service modern customers expect — instant, always-on, personalized and pleasant.
Fraud Detection
With the rise of identity theft and account takeover frauds, traditional authentication methods are no longer sufficient. Artificial intelligence and machine learning enhance security by implementing biometric identifiers such as facial recognition, fingerprint scanning, and voice recognition, which are more difficult for fraudsters to replicate.
The use of machine learning algorithms has been particularly effective, achieving up to 96% accuracy in reducing fraud for ecommerce businesses. These technologies help in identifying patterns and anomalies in large datasets, which is vital for early detection and prevention of eCommerce fraud.
Despite the significant advancements AI and ML are not without flaws. Issues such as false positives and the need for human understanding in certain cases, like reviewing high-value transactions, shows that you can’t do security right without manual reviews.
Dynamic Pricing and Micro-Segment Online Shopping
AI pricing algorithms ingest and analyze data streams instantly to dynamically calibrate optimal prices. It is the purest use of machine learning in e-commerce you can think of. Imagine prices that fluctuate to match fast-shifting signals like:
- Spikes in demand when a product goes viral on TikTok or gets featured by an influencer;
- Sports events, holidays and weather changes affecting seasonal items;
- Competitors running promotions and sales snagging potential customers;
- Individual willingness-to-pay modeled from past purchase patterns.
When demand surges, smart algorithms increase prices to capture more margin from frenzied shoppers. When competitor promotions threaten market share, AI reacts with counter discounts to stay competitive.
It gets better. Dynamic pricing linked to machine learning recommendation engine produces granular micro-segment pricing that helps to customize offers to different shopper groups in a new, elevated way. Budget buyers may see lower prices while premium shoppers get exclusive bundling deals or incentives.
You could never do it by hand. No way, no how.
Optimized Logistics and Inventory Management
So you have used one of the AI recommendation engines, added a trick like visual search, and spruced things up with some more retail AI solutions to reduce your churn and increase conversions. Now your online store gets an increase in orders and everyone is happy… right? Right, but only if your logistics are ready for this ride.
Inventory is one of the biggest costs and biggest headaches for e-commerce companies. Keep too much on hand, and you tie up cash while risking waste. Don’t stock enough, and you miss sales due to out of stock messages.
Machine learning and artificial intelligence are here to save the day again.
Algorithms crunch historical sales data, seasonality patterns and external signals like weather forecasts to accurately predict future demand. This means retailers can dynamically calibrate stock levels to avoid overage and underage. For example, if warm winters are hurting coat sales based on inventory analytics, buys for next season can be adjusted downward to cut costs.
AI also enables real-time visibility and optimization across supply chains. Sensors track inventory at every node, while machine learning solutions reroute items where needed to meet customer delivery expectations.
Applications of Machine Learning: Product Recommendation and other Solutions in Retail
Our goal for this part is to get you back to the reality of cost efficiency, development and implementation strategy when it comes to data and machine learning as well as personalisation in eCommerce. So, we give you the list of ways machine learning and AI can enhance your ecommerce site, and also share what we know about how it works and what it takes to actually get it going.
Recommendation systems
Recommendation engines apply machine learning to data like past purchases, browsing history, product attributes, and ratings to predict each customer’s preferences and recommend relevant items.
There was a big scandal in the US once — the father saw that Target sends baby-related coupons to his teenage daughter and confronted the store managers for inappropriate behavior. They apologized and blamed faulty algorithms. However, algorithms were not at fault — his daughter was actually pregnant. Is it really ok that deep learning departments know more about us and our close ones than we do? No. That is why AI use in eCommerce industry should be regulated.
Recommendation engines use product features, user traffic, and interaction data to provide personalized product suggestions. They can be tailored for specific online stores, with larger stores like Amazon or Alibaba often opting for custom-built solutions. The development cost for such custom engines can quickly escalate to tens of thousands of dollars. Alternatively, there are ready-made add-ons for popular ecommerce platforms like Shopify or WooCommerce, which offer a simpler installation process at a presumably lower cost.
Another option is to use marketing automation platforms or specialized tools compatible with your ecommerce platform, offering a blend of ease of installation and customization options.
AI-Powered Search Tools
When selecting an AI-powered search tool, consider aspects like the types of recommendations (collaborative or content filtering), the quality of recommendations, analytics capabilities, compatibility with your ecommerce platform, and the overall cost and value for money.
Implementing these tools involves data migration, onboarding, and integration with your current system. Vendors often provide support during these processes, but it’s important to inquire about the level and extent of this support.
Some prominent tools in the market include Google Cloud AI and Vertex AI, Algolia, Bloomreach, Clerk, Emarsys, and Nosto. Each offers unique features and integrations, and your choice should align with your business needs and budget. It’s also important to consider the scalability of these tools as your business grows.
Data Enrichment for Better Customer Experience
Data enrichment in ecommerce, facilitated by AI and ML, not only enhances customer data but significantly impacts marketing and user experience. For instance, enriched data can lead to more personalized email marketing campaigns. By understanding customer preferences and past behaviors, AI algorithms can segment customers more effectively, leading to emails that resonate more with each individual, thus improving open rates and engagement.
In terms of UX, enriched data helps in creating a more intuitive and personalized shopping experience. For example, a customer who frequently purchases books in a specific genre on an ecommerce site could be greeted with recommendations for similar books on their next visit. This not only makes the shopping experience more relevant but also increases the likelihood of purchase.
AI-driven data enrichment also enhances retargeting strategies. By analyzing browsing and purchase history, AI can help target ads more effectively to customers who showed interest in certain products but did not make a purchase, thereby improving conversion rates.
In essence, AI and ML-driven data enrichment transforms raw customer data into actionable insights, enabling ecommerce businesses to tailor their marketing efforts and UX to meet individual customer needs, ultimately leading to increased customer satisfaction and sales.
Customer Sentiment Analysis
One viral complaint tweet can tank perceptions. But AI gives ecommerce businesses an early warning system to monitor feelings and doubledown on what shoppers love.
Sentiment analysis AI ingests loads of unstructured feedback from:
- Product reviews
- Social media posts
- Complaint and return forms
- Chatbot conversations
Using natural language processing, it interprets emotions like satisfaction, frustration, excitement and more. Retailers see real-time dashboards analyzing sentiment from most positive to most critical. Leading questions bubble up too. “Why are shoppers disappointed by shipping times?” “What product features are receiving praise?” With deep listening powered by machine learning, even the voice of silent loyalists gets heard.
Chatbots and Virtual Assistants
Chatbots use neural networks trained on human conversational data to understand natural language questions and respond appropriately, providing customer service through dialog. The key is that while terms like machine learning and neural networks sound complex, they are just mathematical functions finding patterns.
AI-powered chatbots and virtual assistants give every customer service on-demand from a personalized shopping companion.
These AI conversational agents can:
- Answer common product questions like pricing, sizing, care instructions etc.
- Provide personalized product suggestions based on purchase history
- Look up order and shipping status for customers
- Guide customers to relevant support articles or contact info
- Seamlessly transfer complex issues to human agents
Chatbots handle high volume basic inquiries to resolve common issues instantly 24/7. This saves service teams for specialized cases.
Virtual assistants take capabilities further with empathetic dialog. They build personal relationships over time by understanding context like past interactions. It’s like having a stylist or advisor ready anytime you need advice.
Over 60% of customers now prefer chatbots and assistants over waiting on hold or digging through FAQs. And AI conversation analytics reveals macro trends plus individual sentiment shifts.
Major eCommerce platforms are already racing to implement as many machine learning applications as possible to beat the competition. We will share their successes next, but it comes with the warning. Personalization in eCommerce is important, but you are not late to the party even if you haven’t done anything about it yet. You have time to make use of machine learning to your benefit. Word.
CTA: Entrust a Google Certified partner with AI-Powered eCommerce Strategy for 2024
Google and Co: The Key Players in AI in eCommerce Field
Google AI Solutions
When selecting an AI-powered search tool for ecommerce, check on Google’s AI products — the best tools created by Google for retail, — including Google Cloud AI and Vertex AI. These platforms offer scalable, robust analytics capabilities and can be integrated seamlessly with various systems.
Google Cloud AI provides machine learning services and APIs for analyzing customer behavior and preferences, which can enhance product search and recommendations.
- Predictive analytics. Google Cloud AI excels in forecasting customer trends and behavior, helping businesses anticipate market changes.
- Automated customer service. Integrates AI chatbots and virtual assistants to provide responsive and accurate customer support.
- Data management and analysis. Offers tools for data warehousing and analytics, enabling businesses to extract actionable insights from their customer data efficiently.
- Personalization at scale. Tailors the customer experience by leveraging AI to personalize marketing messages and product recommendations.
Google Discovery AI
- Enhanced product discovery. Utilizes machine learning to improve product discovery on ecommerce sites, offering personalized recommendations based on user behavior and preferences.
- Dynamic content optimization. Automatically adjusts the content displayed to individual users, enhancing user engagement and experience.
- Real-time analytics. Provides real-time insights into customer interactions, helping businesses to quickly adapt their strategies to changing market trends and consumer needs.
- Seamless integration. Easily integrates with existing ecommerce platforms, making it a versatile tool for a wide range of online retail businesses.
Google Vertex AI. Uses deep neural networks for retail media strategy and planning. It features integrated marketplace insights for tracking influencer impact and automated bidding for large-scale optimization. You get:
- Advanced media strategy. Uses deep learning to analyze consumer behavior, enabling more effective ad placements and content targeting.
- Marketplace insights. Tracks and measures the influence of social media influencers, providing valuable data on campaign effectiveness across various channels.
- Automated bidding. Employs ML for real-time bidding optimization, handling millions of transactions, and adapting bids to market changes instantly.
Google Vertex AI Search and Conversation. It is a sophisticated tool designed with a technical focus:
- Advanced search algorithms. Its search algorithms ensure high accuracy and relevance in search results for complex ecommerce queries.
- Natural Language understanding. Integrates advanced natural language processing capabilities, allowing it to understand and respond to customer queries in a more human-like, conversational manner.
- Real-time data processing. Capable of handling and processing large volumes of data in real time, crucial for dynamic and fast-paced eCommerce environments.
- Custom model training. Provides the ability to train and deploy custom models, enabling tailored search and conversation solutions specific to the unique requirements of an ecommerce business.
If you don’t know where to start with Google Solutions implementation, drop us a message
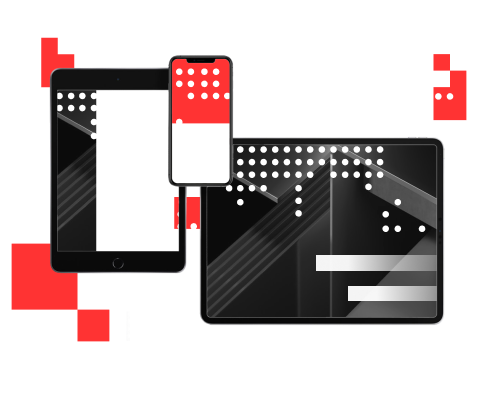
Some extra Google AI functions eCommerce businesses might benefit from:
Cloud Vision. Google’s powerful computer vision tool is capable of understanding product images and scanning documents like purchase orders and invoices. It offers significant efficiencies in merchandising and logistics.
Look to Speak. This innovative tool combines speech technology with Google Lens image recognition to assist shoppers with disabilities. By simply looking at a product through a smartphone camera, users can get details on demand, enhancing accessibility in ecommerce.
Shoploop & Shoppable Video. These platforms integrate AI video recognition and augmented reality for a seamless shopping experience. They allow creators and businesses to tag products in video clips, while viewers can try products virtually, adding a new dimension to online shopping personalization.
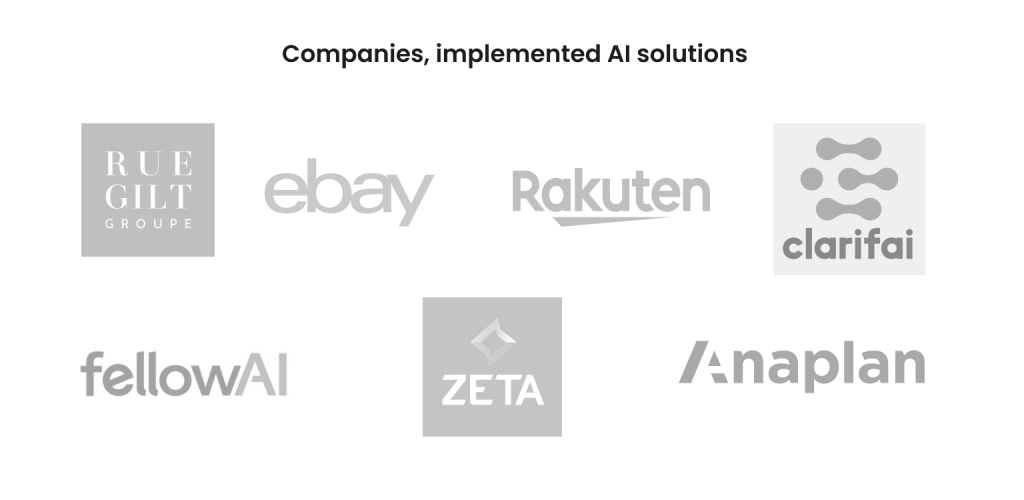
Amazon
Refined algorithms for product recommendations. Amazon’s algorithms suggest complementary products and optimize bundle pricing in real-time. This helps businesses on Amazon by increasing the likelihood of additional purchases through relevant suggestions.
Voice and image search with AI acquisitions. It indicates a push towards more natural search methods. Customers find products using voice or image search much faster.
Personalization engines. Amazon’s AI-driven personalization engines deliver tailored promotions to customers, boosting sales through targeted marketing that resonates with individual preferences and shopping habits.
IBM’s Watson
IBM’s Watson is a powerful AI platform utilized extensively in ecommerce for its advanced data analysis and natural language processing capabilities. Watson provides personalized customer experiences by understanding customer preferences and behaviors. It helps in optimizing supply chain management through predictive analytics and enhances decision-making processes. Additionally, Watson’s AI can improve customer service with its efficient chatbot solutions, offering real-time, accurate responses to customer inquiries. This technology helps ecommerce businesses to make data-driven decisions, streamline operations, and create more engaging customer experiences.
ML Use Cases: More eCommerce Examples
Here are some inspiring machine learning use cases. Don’t think that if you are a small or middle-sized business you can’t follow in some of these mogul’s footsteps. Like any disruptive and massive technology, machine learning and AI in e Commerce spread wide and “down” from high-brow companies to anyone ready to try and implement new solutions and embrace new eCommerce trends.
Rue Gilt Groupe.This luxury merchandise company uses AI and ML to offer personalized product recommendations to its customers. By analyzing a vast catalog of premium brands, their AI system tailors suggestions to individual customer preferences, enhancing the shopping experience
Rakuten. Known as “the Amazon of Japan,” Rakuten heavily invests in AI to understand customer behavior. They analyze their massive product database to predict sales patterns and use AI for product classification and image recognition. Their Fits Me app, which used image-recognition and customer input data to recommend clothing sizes, was a notable advancement in customer satisfaction for online fashion
eBay. eBay employs AI for customer advice, personalized recommendations, and improving various aspects like shipping and buyer-seller trust. In 2019, eBay reported that its AI technology helped identify 40% of online credit card fraud with high precision, showcasing the potential of AI in fraud detection.
Zeta Global. This company combines the power of machine learning and AI with marketing automation to identify potential customers and personalize experiences. By automating marketing activities and applying data insights, Zeta Global helps businesses enhance customer relationships. However, the challenge lies in ensuring that the AI doesn’t over-rely on automated interactions, losing the human touch that can be crucial in customer relationship management.
Clarifai. Clarifai’s AI platform helps in content classification and moderation across various industries, including ecommerce and retail. This AI-powered recognition tool classifies images, videos, audio, and text, but it also incorporates human moderators for added accuracy, acknowledging the limitations of AI in understanding nuanced content.
Anaplan. Anaplan’s AI platform assists retailers in customer retention and acquiring new customers by analyzing real-time data from websites, social media, and other sources. It uses predictive data for customer recommendations and forecasting business outcomes. The challenge for Anaplan and similar platforms is to balance data-driven insights with the unpredictability of human behavior.
Fellow AI. This company uses image recognition for real-time inventory management. Lowe’s, a home improvement retail chain, uses Fellow robots (“LoweBots”) in some locations to assist customers and monitor inventory in real time. While these AI solutions increase efficiency, they also raise concerns about the potential reduction in human jobs due to automation.
The number of machine learning in retail use cases probably is growing exponentially, because AI tools become more accessible, cheap, and simple to implement. It now just takes a decision to implement machine learning in your eCommerce business. Well, it takes money and time too, but not much more than any other automation/digitalisation tools.
10 Machine Learning & AI Related Terms Useful for eCommerce
You don’t need to know how microchips work to use your smartphone. You don’t have to understand what neural networks are to optimize your online retail business using AI. However, knowing what you are working with helps you get more out of it. We’ve prepared a small urban AI and ML dictionary, linking it to potential business growth for ecommerce stores.
Just refresh these terms in your memory before you start planning for personalized product discovery and google retail search in 2024.
Artificial Intelligence (AI) is the broad concept of machines displaying human-like intelligence to perform tasks like visual perception, speech recognition, and decision-making. The umbrella term for more specific approaches like machine learning.
Machine learning is a subset of artificial intelligence that allows computers to learn patterns from data without explicit programming. Algorithms identify signals in data that correlate with desired outcomes to make predictions or decisions. For example, it can correlate product characteristics and past purchases to provide AI powered recommendations to your clients.
Neural networks are inspired by the human brain, these consist of different layers of algorithms that each detect multiple signals from the data. The layers then weigh and combine these signals, becoming more accurate through continuous learning on new data. They power capabilities like product image recognition.
Deep Learning: A complex form of machine learning based on artificial neural networks, inspired by the human brain’s interconnected web of neurons transmitting signals. Useful for product image analysis and classification.
Supervised learning: A machine learning technique where algorithms are first trained on extensively labeled datasets that define the “right answers,” allowing the system to learn the rules that map different inputs to the target variable. Enables chatbots.
Unsupervised learning: Algorithms are let loose on raw, unlabeled data sets to find subtle patterns and groupings without pre-defined categories or rules. Can reveal unexpected connections between products.
Reinforcement Learning: Here algorithms learn optimal behavior through trial-and-error interactions with an environment based on positive or negative feedback, like a gamified approach to chatbot training.
Computer vision is based on neural networks that analyze millions of product images to recognize patterns and properties, allowing AI cameras to identify items on shelves and enable cashier-less stores.
Generative AI is a class of AI algorithms focused on using machine learning models to generate new, synthetic content like images, text, audio, and video that are similar but not identical to their training data. In the eCommerce business, generative AI is used for product images and listings, design and creatives, chatbots and recommendations, and demand forecasting.
Conversational AI (a little cousin of Gen AI) is basically talking Turing test robots ready to field every customer question 24/7. No more salaries wasted on idle sales clerks? We have warned you from the beginning that eCommerce AI solutions come with layoffs. There is just no way around it.
Now, please, let’s take a moment and talk about responsibility.
How to Use Machine Learning and Artificial Intelligence Ethically
Personalization through a recommendation system, a major benefit of AI, can sometimes accidentally lead to exclusion. For instance, if AI recommends products based on past purchases, it might overlook new or diverse interests of the customer. Imagine a scenario where an AI system, trained mostly on data from young urban consumers, might not effectively cater to or recommend products relevant to older, rural customers.
Accessibility is another crucial area. AI can make shopping easier for everyone, including people with disabilities. Voice-assisted shopping, for example, is a boon for visually impaired users, allowing them to shop online independently. However, if these AI tools aren’t designed with diverse needs in mind, they might not be as helpful for everyone. Which means when you hire and instruct your developers and QA, make sure the team is diverse or at least has access to large amounts of data on the subject.
Privacy and data ethics are also big concerns. AI systems collect lots of data to make shopping easier and more personalized. But this raises questions about how that data is used and protected. Consumers worry about their personal information being misused or falling into the wrong hands.
Lastly, there’s the issue of job displacement. AI can do some jobs faster and cheaper than humans, but this also means that some people might lose their jobs to machines. We have actually started the article with this prediction. This raises ethical questions about the balance between technological advancement and its impact on the workforce.
In summary, while retail AI solutions bring many positives to ecommerce, it’s important to think about these broader impacts on society, ensuring that technology is used responsibly and benefits everyone.
- Benefits of AI and Machine Learning in eCommerce Business
- Applications of Machine Learning: Product Recommendation and other Solutions in Retail
- Google and Co: The Key Players in AI in eCommerce Field
- 10 Machine Learning & AI Related Terms Useful for eCommerce
- How to Use Machine Learning and Artificial Intelligence Ethically
Planning to improve development process?
Decrease the entropy of product design and development, and increase your chances of success.
Written by
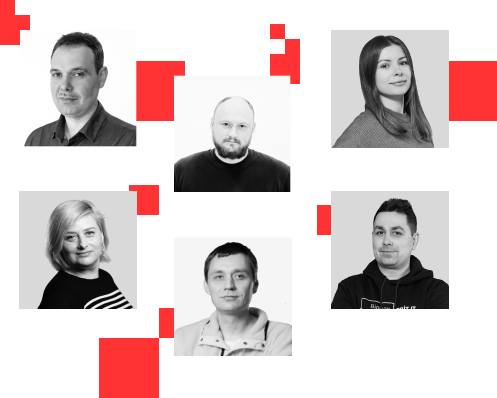
Hire a team
Let us assemble a dream team of specialists just for you. Our model allows you to maximize the efficiency of your team.
We Help With
Your tech partner needs to be well versed in all kinds of software-related services. As the software development process involves different stages and cycles, the most natural solution is to have them all performed by the same team of experts. That’s exactly what our diverse range of services is for.
The choice of technology for your software project is one of the defining factors of its success. Here at QArea, we have hands-on experience with dozens of popular front-end, back-end, and mobile technologies for creating robust software solutions.
In-depth familiarity and practical experience with key technologies are one of the cornerstones of successful software development and QA. But it also takes specific knowledge of the industry to develop a solution that meets the expectations of the stakeholders and propels its owner to success.

Ensure an effective online presence for your business with a corporate site.