Machine Learning in Healthcare: Hope over Hype in Patient Care
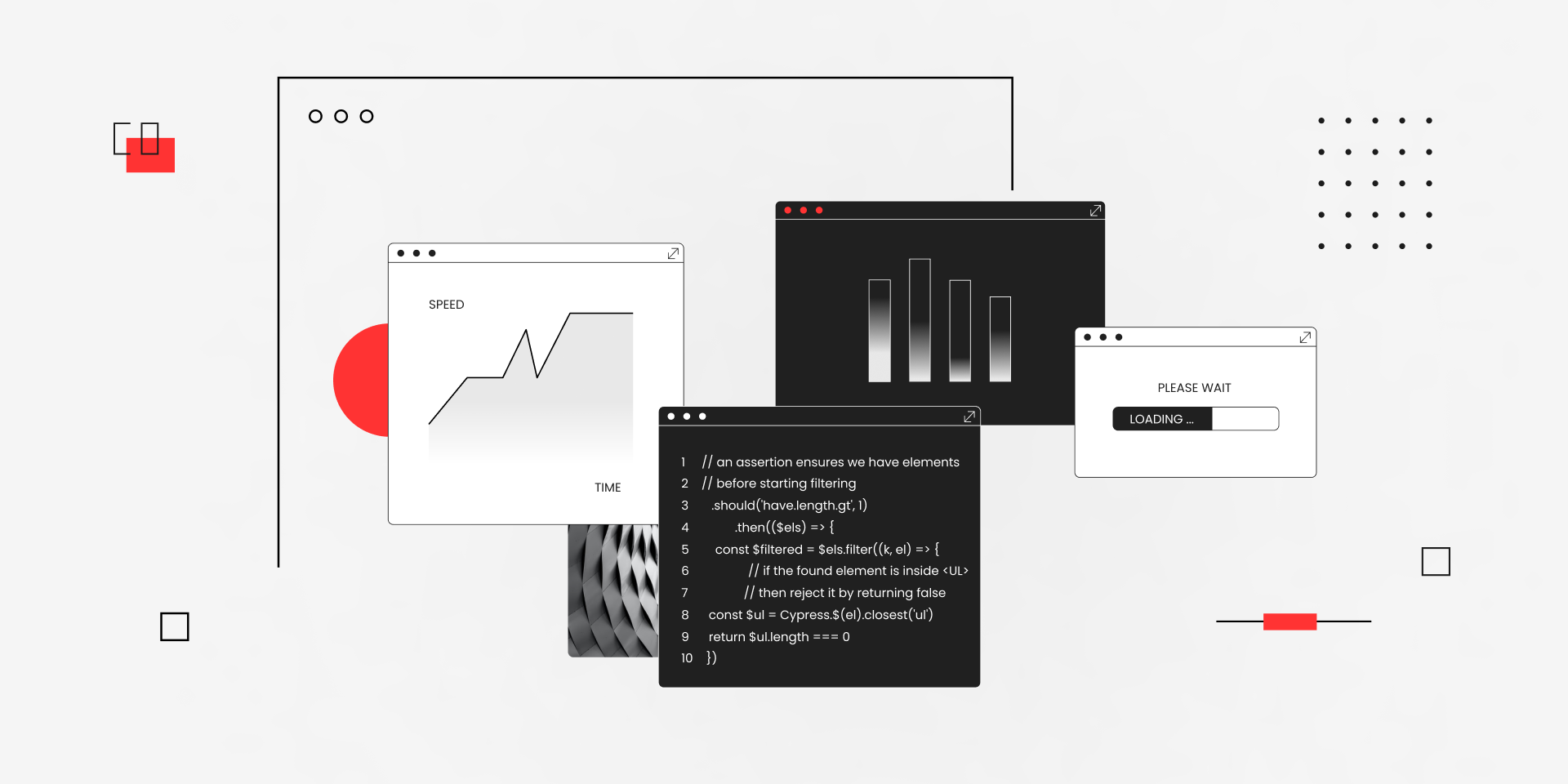
We care about machine learning for healthcare, not because it is a new development toy that tickles our geeky brains and comes with wealthy clients. We care, because it gives hope. It gives hope for a better tomorrow to those who need it most.
And above all, it saves lives already.
Just a few use cases of machine learning in healthcare that makes our team feel better about the world:
- AI accurately assesses liver lesions 95% accuracy, improving oncology care;
- Machine learning-based software predicts heart failure readmissions with top precision;
- AI-driven drug discovery gives hope to patients with neurodegenerative diseases
- Machine learning aids pathologists in quicker, more accurate cancer diagnoses;
- Cloud-based AI improves early detection of heart rhythm disturbances in remote cardiac care;
This article wouldn’t happen if not for our partners and good friends from Amazinum — a Data Science company that connects ML and AI professionals with projects in need of innovation.
They share our hopes, concerns and ethical approach to using ML and deep learning to increase efficiency of healthcare, be it development or automation. They’ve also shared quite a few insights on how AI-based tech can help healthcare evolve while staying beneficial for all the parties involved.
Application of Machine Learning: Why Healthcare? Why Now?
Healthcare is drowning in data. We’re talking petabytes of patient records, medical images, and genomic sequences. It’s a gold mine for machine learning, but it’s also a massive headache for healthcare providers. They’re struggling to make sense of it all, and that’s where ML comes in.
ML in healthcare algorithms can churn through this massive amount of data faster than any human could. They can spot patterns, make predictions, and uncover insights that would take years for a team of experts to find.
This makes such algorithms powerful tools for diagnosis, treatment planning, and patient management. And that’s why healthcare is the perfect playground for ML.
But why now? Because we can’t afford to wait.
- Healthcare costs are through the roof, and everyone’s feeling the pinch. Patients, providers, and payers all want better outcomes at lower costs.
- Chronic diseases are on the rise, and people are living longer. That means more complex care and higher costs.
- We’ve gotten better at keeping people alive longer, but neurodegenerative diseases like Alzheimer’s and Parkinson’s are turning those extra years into a struggle rather than a victory.
- There’s a massive shortage of healthcare professionals, especially in rural and underserved areas.
- Patients are demanding more personalized, convenient care. They want to be treated like individuals, not numbers.
- The technology is finally ready for prime time. We have the computing power, deep learning algorithms, and the data to make machine learning models work in healthcare.
- Investors are taking notice of the potential for ML in healthcare. An aging population with more disposable income means a growing market for innovative healthcare solutions.
Of course, there are challenges. Healthcare data is notoriously messy and siloed. There are privacy and security concerns. And there’s always the risk of bias and data-based errors in ML models. But that’s where collaboration comes in. We need healthcare providers, tech companies, researchers, and regulators to work together to get this right.
But first, let’s enjoy our take on the advantages of ML in healthcare.
Ready to join the next wave of healthcare innovation?
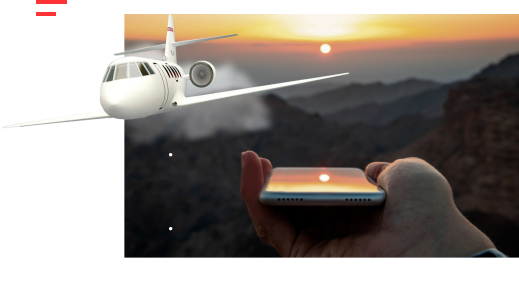
18 Use Cases and Benefits of Machine Learning in Healthcare Industry
There’s no shortage of hype around ML in healthcare. But the truth is, when it’s done right, ML can be a game-changer. Here are some of the ways it’s already making a difference. We warn you — there are SO MANY of them.
(Wait, don’t quit just yet. Maybe it’s Monday morning, and reading all 18 ML applications in healthcare seems like too big of a drag. We’ve put a summarizing table there for that reason exactly.)
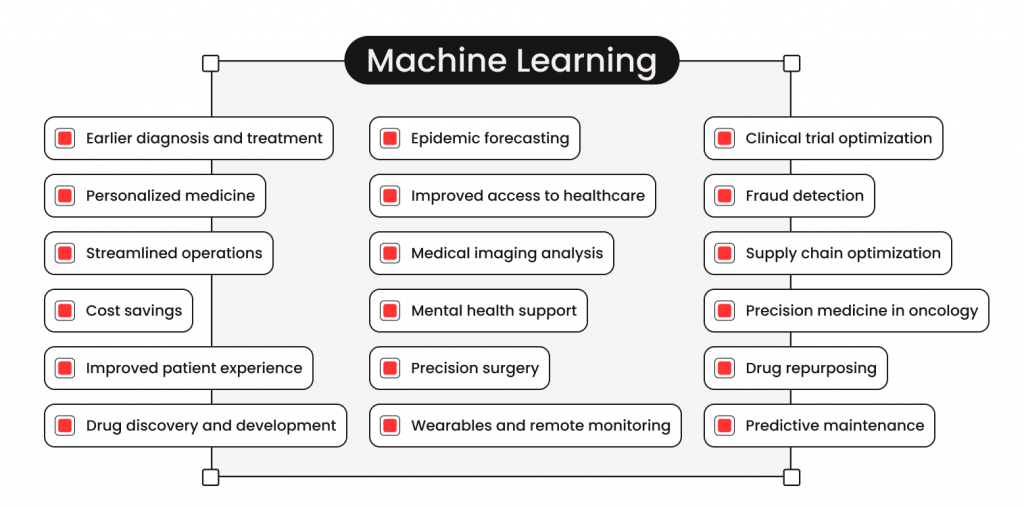
Earlier diagnosis and treatment
ML algorithms can spot early signs of disease that humans might miss. Take cancer, for example. ML can analyze medical images and identify tiny tumors before they spread. That means patients can get treated sooner, when the odds of survival are better. It’s not just cancer, either. ML is being used to detect everything from heart disease to diabetic retinopathy.
“There are obvious patterns in some diseases and making a model to study them can help predict the development of illness and prevent specific unpleasant symptoms even before they occur at a minimal level. Computer vision combined with advanced image analysis is one of the keys to success here. It can be used to detect early signs of skin changes that may lead to melanoma or detect almost invisible signs of lung cancer based on the dark-light patterns even at the simplest scans.” — Amazinum
Personalized medicine
One-size-fits-all treatments are so last century. ML can help providers tailor care to each patient’s unique genetic profile, medical history, and lifestyle factors. Algorithms in healthcare can predict which treatments are most likely to work for each individual patient. That means better outcomes and fewer side effects.
Streamlined operations
Healthcare is a complex, fragmented system. ML allows healthcare facilities to automate routine tasks and optimize resource allocation, reduce waste and improve operational efficiency. For example, machine learning algorithms can predict which patients are most likely to miss appointments, so healthcare practitioners can proactively reach out and remind them.
“There are many processes that just drain attention and resources — routine, continuous, multi-level. Often, the only way to approach them efficiently and without tearing everything down, is from the data-science stand point. Before you implement any automation, you get to see which “process conjunctions,” which part of the flow should be prioritized. It allows using new tech to increase efficiency at a fraction of a price and time needed to redo the entire system.” — Amazinum.
Cost savings
Healthcare is expensive. Some people lose their houses to cover medical bills. But ML can help bend the cost curve.
By reducing readmissions, preventing complications, helping all the sides to make informed decisions, and optimizing treatment plans, ML can save providers and payers millions of dollars. Plus, by catching diseases earlier and keeping patients with high life expectancy healthier, ML can reduce the overall burden on taxpayers.
Improved patient experience
AI-driven solutions and ML models can help providers deliver care more quickly and conveniently. For example, chatbots and virtual assistants can triage patients and answer basic questions, freeing up human staff to focus on more complex cases. And remote monitoring devices can use ML to detect changes in a patient’s condition and alert providers before a crisis occurs.
If honestly, new tech allows healthcare organizations to take care to such a new level that it deserves a separate article. One thing we are sure about is that real-time data processing and machine learning for healthcare will define how we see and experience the entire healthcare system from now on.
“ML in healthcare allows us to reduce mistakes caused by a human factor. Doctors, pathologists, and lab personnel can get tired, or bored, or be overwhelmed by personal problems and simply miss what’s important, misdiagnose a patient, skip some important information like allergies or side effects of complex drug prescriptions. Data science models are also not without fault, but their discrepancies are minimized and they learn from mistakes much more efficiently than humans.” — Amazinum.
Drug discovery and development
Bringing a new drug to market is a long, expensive process. Healthcare machine learning speeds things up by predicting which compounds are most likely to be effective and safe. By analyzing molecular data, ML algorithms can identify promising drug candidates and optimize their design. Faster, cheaper, and more targeted therapies for patients enter the scene.
Epidemic forecasting and management
ML can help public health officials predict and respond to disease outbreaks. Checking social media, search engines, and other sources, ML algorithms can detect early warning signs of an epidemic and forecast its spread. That means health authorities can allocate resources more effectively and take steps to contain the outbreak before it spirals out of control.
Improved access to healthcare
Machine learning for healthcare in wealthy countries is mostly about improving what already works. For example, healthcare monitoring can be now done almost unnoticeable for patients which both levels up their comfort and allows for early detection of the most widespread problems like cardio-vascular diseases.
More importantly, using machine learning can improve healthcare delivery in third-world countries that lack access to modern diagnosis, research and treatment.
Medical imaging analysis
ML is revolutionizing the way we analyze medical images. By training on vast datasets of X-rays, CT scans, and MRIs, ML algorithms can detect subtle abnormalities that human radiologists might miss. For example, ML can identify early signs of lung cancer on chest X-rays, detect brain tumors on MRI scans, and diagnose skin cancer from photographs. That means earlier detection, more accurate diagnoses, and better outcomes for patients.
“The ability of ML and AI tools to work with imperfect visual data and constantly improve in analyzing it helps service communities that don’t have access to state-of-the-art scanning machines. And it is more than half of the world.” — Amazinum.
Mental health support
Mental health is a growing concern, but many people still struggle to access care. ML can help bridge the gap by providing personalized support and monitoring. For example, ML-powered chatbots can provide cognitive behavioral therapy and track a patient’s progress over time. And ML algorithms can analyze social media posts and other data to identify individuals at risk of suicide or self-harm.
Precision surgery
ML is transforming the way surgeons operate. By analyzing medical images and other data, ML algorithms can help surgeons plan and execute procedures with greater precision. For example, ML can guide robotic surgery systems to make more accurate incisions and avoid critical structures. That means fewer complications, shorter recovery times, and better outcomes for patients.
Wearables and remote monitoring
ML is powering a new generation of wearable devices and remote monitoring systems. By analyzing medical data from sensors and other sources, ML algorithms can track a patient’s vital signs, activity levels, and other health indicators in real-time.
Providers can detect potential problems early and intervene before they become serious. For example, ML-powered wearables can alert patients with heart disease to changes in their cardiac rhythm, while remote monitoring systems can help people with diabetes manage their blood sugar levels more effectively.
“One of our latest projects was related to the analysis of data that comes from sensors. Just so you can imagine — the system in question writes down around 300 markers per second. With a 24-hour patient observation period, it comes to almost 26 million data entries per day. We have created a classification system focused on spotting paranormal activities and trends that can be later analyzed by human physicians.” — Amazinum
Clinical trial optimization
Clinical trials are essential for bringing new treatments to market, but they’re often slow and inefficient. ML can help optimize trial design and patient recruitment. By analyzing electronic health records and other data sources, ML algorithms can identify patients who are most likely to benefit from a particular treatment and match them with appropriate trials. This way, we get faster, more targeted trials and quicker access to new therapies.
Fraud detection
Healthcare fraud is a billion-dollar problem. Artificial intelligence and machine learning can help spot suspicious patterns and anomalies in claims data that might indicate fraud. By analyzing provider billing patterns, patient demographics, and other factors, ML algorithms can flag potential cases of fraud for further investigation. That means more resources for legitimate care and less waste in the system.
“A somewhat simple example, but still fraud-relevant. We’ve worked on a visual analysis system that determined the validity of a self-administered express COVID test. People massively downloaded pictures of positive/negative express tests or shared pictures done from different angles with coworkers or subscribers in special groups. A rather simple ML model allowed our clients to identify fraudulent test result pictures.” — Amazinum.
Pharmaceutical supply chain optimization
Getting the right drugs to the right patients at the right time is a complex logistical challenge. Machine learning for healthcare can help streamline the pharmaceutical supply chain by predicting demand, optimizing inventory levels, and reducing waste. By analyzing data on prescribing patterns, patient demographics, and other factors, ML algorithms can help drug manufacturers and distributors anticipate shortages and ensure a steady supply of essential medicines.
“Pharmacies benefit greatly from top tools data science has to offer. Automation and data science implementation may seem overwhelming, but we always recommend to go about it step-by-step and start with bite-sized projects, scaling solutions when possible and necessary:
Short term projects — detection and recognition of drugs by implementing natural language processing, computer vision, etc.;
Middle-team projects — expanding one-module solutions to different tasks and processes in one or more pharmacies;
Long-term projects — not only detecting and fixing different drugs, medical tools and supplements stored, but also programming logistics analysis, creating complex analytical dashboards, launching recommendation and prediction systems.” — Amazinum.
Precision medicine in oncology
Cancer is a complex and heterogeneous disease, and traditional one-size-fits-all treatments often fall short. ML is enabling a new era of precision medicine in oncology, digging in genomic, clinical, imaging and general patient data to develop personalized treatment strategy. By identifying the specific genetic mutations driving a patient’s cancer, ML algorithms can predict which targeted therapies are most likely to be effective. That means more precise, less toxic treatments and better outcomes for patients.
“Data science helps to deal with the challenges of nanomaterial complexity. Machine learning systems simplify the use of nanotechnology to improve medicine delivery. This tech allows tiny particles to get medicine right where it’s needed most. It can make treatments, like for cancer, more effective and safer, with fewer side effects and more predictable healthcare outcomes” — Amazinum.
Drug repurposing
Developing a new drug from scratch can take years and cost billions of dollars. Machine learning application is helping researchers identify existing drugs that could be repurposed for new indications. By analyzing vast amounts of molecular, clinical, and pharmacological data, ML algorithms can predict which drugs are most likely to be effective against a particular disease.
Predictive maintenance for medical devices
Medical devices like MRI machines, ventilators, and infusion pumps are essential for modern healthcare, but they’re also expensive and complex. ML can help predict when these devices are likely to fail, so maintenance can be scheduled proactively. By analyzing data on usage patterns, error codes, and other factors, ML algorithms can identify potential problems before they cause downtime or patient harm. That means less disruption to clinical workflows, lower maintenance costs, and better patient safety.
Discover how ML can transform your healthcare solutions for better patient outcomes!
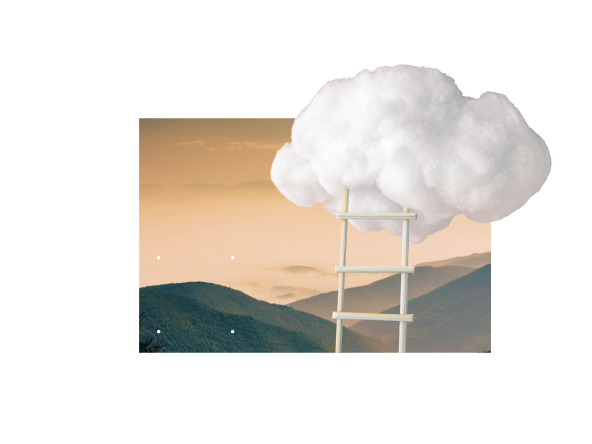
Artificial Intelligence and Machine Learning for Healthcare Most Pressing Needs
ML Tools in Healthcare
Predictive Analytics | |
Diagnostic Assistance | – Early detection of diseases. – Accurate prediction of disease progression. |
Patient Care | – Anticipating patient needs. – Tailoring care plans based on patient data |
Administrative Efficiency | – Predicting patient admission rates. – Resource allocation optimization. |
Research and Drug Development | – Identifying potential drug targets. – Analyzing clinical trial data for insights. |
Data Analysis and Management | Forecasting healthcare trends. |
Natural Language Processing (NLP) | |
Diagnostic Assistance | – Interpreting patient records and medical notes. – Assisting in differential diagnosis. |
Patient Care | – Enhancing communication with patients via chatbots. – Extracting patient information from unstructured data. |
Administrative Efficiency | – Automating documentation and reporting. – Streamlining communication between departments. |
Research and Drug Development | – Mining scientific literature for research insights. – Automating data entry in research documentation. |
Data Analysis and Management | – Sentiment analysis in patient feedback. – Structuring unstructured data for better analysis. |
Image Analysis | |
Diagnostic Assistance | – Enhanced accuracy in radiology and pathology. – Detection of anomalies in medical imaging. |
Patient Care | – Monitoring patient conditions through image tracking. – Assisting in surgical planning with visual data. |
Administrative Efficiency | Reducing diagnostic errors and associated costs. |
Research and Drug Development | – High-throughput screening in pharmaceutical research. – Morphological analysis in biomedical research. |
Data Analysis and Management | Processing large volumes of imaging data efficiently. |
Robotics and Automation | |
Diagnostic Assistance | – Assisting in complex surgeries. – Enhanced precision in medical procedures. |
Patient Care | – Automated monitoring of patient vitals. – Robotics in rehabilitation and patient assistance. |
Administrative Efficiency | – Automating routine tasks like sanitization. – Streamlining logistical operations in hospitals. |
Research and Drug Development | – Automated laboratory processes for drug development. – Precision in experimental procedures. |
Data Analysis and Management | – Handling large-scale repetitive tasks. – Automating data entry and extraction. |
Deep Learning Algorithms | |
Diagnostic Assistance | – Identifying patterns in complex medical data. – Improving accuracy of diagnostics over time. |
Patient Care | – Personalized medicine based on deep patient data analysis. – Predictive models for patient outcomes. |
Administrative Efficiency | Analyzing operational data to identify inefficiencies. |
Research and Drug Development | – Drug discovery and genomics research. – Modeling complex biological systems. |
Data Analysis and Management | Advanced data mining and pattern recognition. |
How to Use ML Whether You’re a Big or Small Business
Now, we know what you’re thinking. “ML is just for the big players with deep pockets and armies of data scientists, right?” Wrong. The beauty of ML is that it can be tailored to fit any size organization, from the mom-and-pop shop to the multinational conglomerate.
Sure, the scale and complexity of the challenges may differ, but the core principles remain the same. It’s all about leveraging data to make smarter, faster decisions that improve patient outcomes and streamline operations. And trust us, every healthcare business has data – whether it’s a handful of patient records or a vast data warehouse.
Consider a small pharmacy. ML can help predict what’s going to fly off the shelves and what’s going to collect dust. It’s a straightforward solution but can make a real difference in cutting down waste and keeping customers happy.
A mid-sized clinic can use an ML-based appointment scheduling system to optimize patient flow and reduce wait times. It’s not just about automation; it’s about making systems smarter to handle growing complexity.
Enterprises face the challenge of scale but also have the resources to experiment. They can afford to set up ‘start-up like’ departments focusing on innovating with AI and ML. Consider a large hospital chain experimenting with ML for predictive diagnostics. They might start in one department as a pilot project. The key here is experimentation and innovation, using a small segment of the business to test, learn, and then scale solutions across the organization.
“Whether it’s a small business or a large enterprise, the key to successfully integrating ML is to tailor solutions to your specific needs and scale. It’s not a one-size-fits-all approach. Understand your unique challenges, start small, experiment, and scale progressively.” — Amazinum
Check on the list of tools many companies use in order for healthcare professionals to make the most of their research, development and interactions with patients.
Who Can Make Those Machine Learning Algorithms Work for You?
So, who will mastermind and implement all these wonders for your company? As always, there are options, and the answer widely depends on your goal and resources.
In-house specialists. If you have a dedicated data science team within your organization, they can work closely with your domain experts to develop custom ML solutions tailored to your specific needs. However, building an in-house team can be costly and time-consuming, and may not be feasible for smaller organizations with limited resources. Big companies also often opt-out of this approach to minimize HR-related costs and onboarding headaches.
Data Science adepts, like Amazinum.
You have an idea that you’d like to implement, but you are not sure whether it is even plausible to achieve the results you want. You may request a dedicated data scientist for your team to work with hundreds of distinct data points and figure this out. Another option — outsource the project at its MVP stage to a company focused on such tasks. It will have both experience and a set of working tools to test your hypothesis and offer the most practical solutions.
“For example, a website that sells healthcare products, be it an online pharmacy or a supplement marketplace, decides to implement a recommendation system. It is proven that such systems if done right increase clients’ satisfaction and boost profits. The same works for medical services portals — even there you can nudge a user to consider a more tailored package.
It takes us about 3 months to create such a system customized to clients’ needs and later about 3 months for the system to peak its efficiency. All data science solutions work with big data volumes at a learning stage and later need some time to unfold and scale in real-life conditions.
Scalability and self-teaching are inherent to such solutions. We make them see trends, and seasonality and learn continuously. This way, what the system recommends on the first days after launch and what the system recommends in 3 months will be different.”
Early adoption of such solutions gives great upper hands compared to competition.” — Amazinum
Full-scale outsourcing development companies, like QArea. When you’ve got a project that needs a mix of heavy-duty development, rigorous testing, and some serious machine learning chops, teaming up with a full-service outsourcing company might just be your best bet.
We can organize the entire development process from the start, or take over the project at any stage. For data science magic we partner with niche expert companies like Amazinum, so our clients get a package deal. Time is saved, cost overheads are cut, and the product gets the best of both worlds — organized development and top AI and ML expertise.
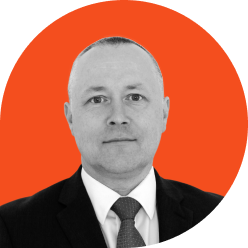
“Healthcare startups tend to forget about regulatory costs. They want to deliver as fast as possible. However, you just need to follow changes in regulations and, if you are outsourcing, choose a company with strong domain expertise. They will have a roadmap on regulatory requirements and how much it will cost to develop and operate within legal guidelines.”
Enhance your healthcare services with AI
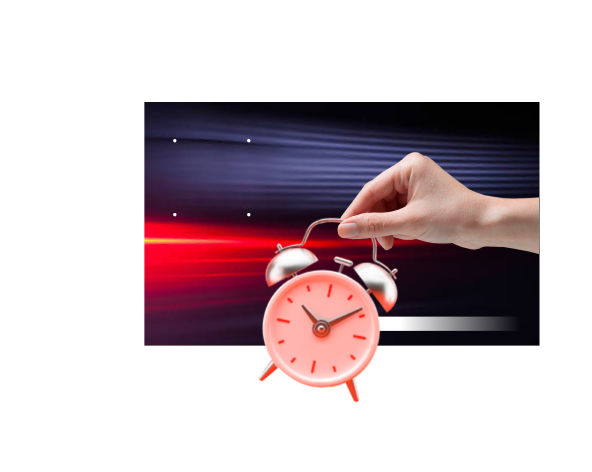
Machine Learning and Artificial Intelligence Tools in Healthcare
Category | Tools/Technologies |
Data Management and Integration Tools | – Electronic Health Record (EHR) systems – Data warehousing and data lakes- Health Information Exchanges (HIEs)- Fast Healthcare Interoperability Resources (FHIR)- Data cleaning and preprocessing tools |
Machine Learning Frameworks and Libraries | TensorFlow, PyTorch, Scikit-learn, Keras, Apache MXNet |
Cloud Computing Platforms | Amazon Web Services (AWS), Google Cloud Platform (GCP), Microsoft Azure, IBM Cloud |
Big Data Processing Tools | Apache Hadoop, Apache Spark, Apache Kafka, Apache Cassandra |
Visualization and Explainable AI Tools | Tableau, QlikView, LIME (Local Interpretable Model-Agnostic Explanations), SHAP (SHapley Additive exPlanations) |
Federated Learning and Privacy-Preserving Tools | PySyft, TensorFlow Federated, OpenMined, Differential Privacy libraries |
Specialized Healthcare ML Tools and Platforms | Google Cloud Healthcare API , AWS Healthcare AI, IBM Watson Health, Microsoft Azure for Healthcare |
Model Deployment and Monitoring Tools | Docker and Kubernetes, MLflow, TensorFlow Serving, Prometheus and Grafana |
Collaboration and Version Control Tools | Git and GitHub, JupyterLab and Jupyter Notebooks, Databricks Collaborative Notebooks, W&B (Weights and Biases) |
Regulatory Compliance and Security Tools | HIPAA-compliant cloud storage, Anonymization and de-identification tools, Blockchain for secure data sharing,Audit logging and access control tools |
Is There Something Left to Develop? Oh Yes, There Is
While reading about machine learning in healthcare, you might think every possible idea is already taken, but that’s far from the truth. It might look like a crowded space, but there are still many areas left unexplored. Here’s where we can still make a big impact:
Rare diseases need attention. Current ML models excel in common conditions, but we’re still in the early stages of effectively predicting and diagnosing rare diseases. Developing ML tools that can analyze patterns in limited data sets specific to rare conditions could be life-changing for many patients.
Mental health deserves more. There’s a need for much more sophisticated ML solutions that can provide deeper insights into mental health conditions, predict crises, and offer real-time, personalized intervention strategies. An estimated 703 000 people die by suicide worldwide each year. We have to do something about it.
Healthcare coaching can be almost free and accurate. There’s potential for AI-powered virtual health coaches that can provide personalized diet, exercise, and wellness advice, especially for chronic disease management, tailored to individual patient data. At some point group appointments allow consulting low-income patients with similar conditions (heart diseases, diabetes). AI coaches are the next level.
Diagnostic tools should be as non-invasive as possible. Using ML for clinical research and developing advanced non-invasive diagnostic tools, like analyzing voice patterns or skin images, could make diagnostics more accessible and less intimidating.
Taking care of health beyond the hospitals. ML tools that can effectively incorporate social and environmental factors into health risk assessments could lead to more holistic approaches to patient care. Doctors listen to you like what… 11 seconds? AI can look at your whole life, not just your medical chart, to figure out health risks.
Drug mix-ups? No more. Developing sophisticated models for machine learning can also revolutionize pharmacology. Such models can then predict drug interactions more accurately, especially considering the individual’s unique genetic makeup.
Smarter hospitals for smarter care. Tools that can predict healthcare resource needs, from staffing to equipment, could greatly improve the efficiency and readiness of healthcare facilities.
Making data ACTUALLY safe. As data sharing is crucial for ML, creating more robust privacy-preserving techniques for secure and ethical data usage is essential.
AI for better healthcare policies. Seriously, have you seen what humans do with those? AI tools that assist in healthcare policy making, predicting public health trends, and planning healthcare infrastructure are needed to address the broader challenges in the healthcare system. We may not even know about those challenges now — but they are there.
Local answers for global health. Developing ML tools tailored to specific geographical and cultural contexts, especially for tackling health issues in underdeveloped regions, is a significant unmet need. This “cultural context” may be a region, or it may be a health condition specific for a certain group. When will doctors FINALLY stop getting confused about creatinine levels of black patients?
Care to create a new AI-driven healthcare solution?
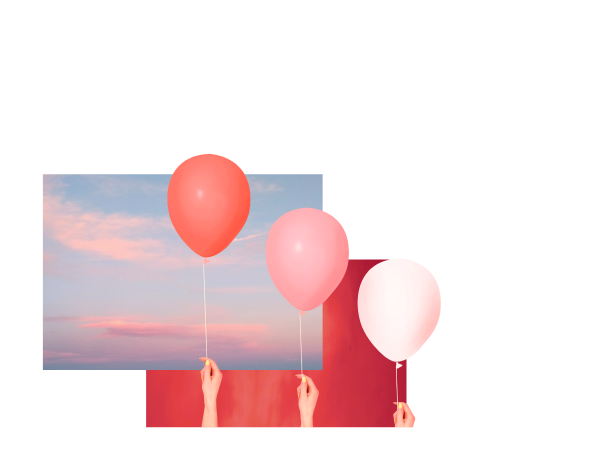
Challenges of Artificial Intelligence Used in Healthcare
The potential benefits of machine learning and artificial intelligence for healthcare services are enormous, but so are the challenges. And we’re not just talking about some abstract, high-level issues that only policymakers and academics care about. We’re talking about real problems that affect everyone involved in the healthcare sector — from the medical moguls at the top to the patients at the bottom.
If you’re a developer or data scientist working on ML in healthcare, we know how you feel. You’re the ones in the trenches, trying to make sense of the mess that is healthcare data collected by profit-focused pharmaceutical companies, mismatched and anxious trial participants, and various healthcare institutions in general.
If you’re a healthcare provider, you’re probably equal parts excited and terrified by the prospect of ML. On the one hand, it could make your job a lot easier and help you provide better care to your patients. On the other hand, you’re probably worried about the liability issues and the potential for unintended consequences. And let’s not forget the workflow disruptions and the need for constant training and upskilling.
And if you’re a patient, just accidentally reading this piece, you might be wondering what the hell any of this has to do with you. But trust us, you have a stake in this. A BIG one. ML algorithms are going to be making decisions that directly impact your care — everything from what treatments you receive to how much you pay for insurance. You need to be informed and engaged every step of the way.
In the rest of this section, we’ll cover the specific challenges facing ML in healthcare, from the technical to the ethical to the organizational. We’ll talk about what’s being done to address these challenges and what still needs to happen.
Technical Challenges of Machine Learning in the Healthcare Industry
Let’s start with the basics: data. It’s the lifeblood of any ML system, but in healthcare, it’s often more like a bloody mess. Electronic health records (EHRs) are notoriously incomplete, inaccurate, and inconsistent. One study found that up to 50% of EHR data is either missing or incorrect. That’s like trying to build a skyscraper on quicksand.
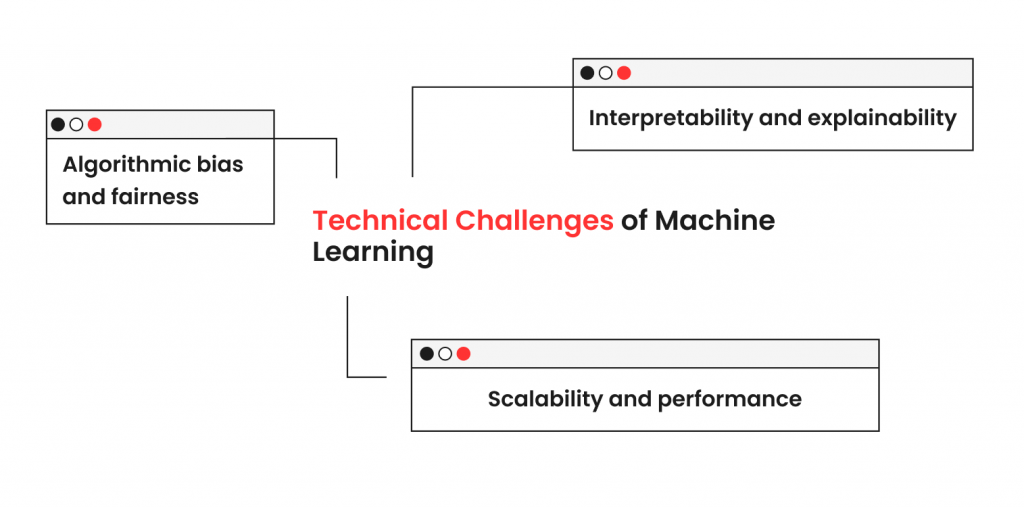
And even when the data points are there, they are often scattered across different systems and formats. Hospitals use different EHR vendors, labs use different reporting standards, and don’t even get us started on the state of interoperability in healthcare. Trying to integrate these large amounts of data is like herding cats — if the cats were also on fire and spread across multiple continents.
Algorithmic bias and fairness
But let’s say you manage to get your data house in order. You’ve cleaned it, integrated it, and fed it to your shiny new ML algorithm. Congratulations, you’ve just inherited a whole new set of problems. Because here’s the thing: ML algorithms are only as unbiased as the data they’re trained on. And healthcare data is far from unbiased.
Take race, for example. It’s a known fact that certain minorities, particularly Black Americans, have been systematically underrepresented in clinical trials and other medical research. That means the data used to train healthcare ML algorithms is often skewed towards white, middle-class patients. The result? Algorithms that perform worse on underrepresented groups, potentially leading to misdiagnoses, inappropriate treatments, and worse outcomes.
And it’s not just race. Gender, age, socioeconomic status — all of these factors can introduce bias into healthcare data. Detecting and mitigating these biases is a huge challenge, especially when you’re dealing with complex, high-dimensional data sets. It requires a deep understanding of both the data and the underlying social and cultural factors that shape it.
“Programmers often say “garbage in, garbage out.” We say “bias in, bias out.” It is our top priority to use different tools and latest best practices to reduce bias in the data sets we work with and pay extra attention to the data sources.
For example, ML models are prone to overfitting. They overly focus on data seen during the training period and may act rigid when introduced to new real-life data in daily operations. We make multi-level tests using new segments of data to detect and reduce overfitting and increase accuracy of our clients’ models.” — Amazinum
Interpretability and explainability
Even if you manage to build a close-to-unbiased algorithm, you’re not out of the woods yet. Because healthcare is a high-stakes domain, and people need to understand how decisions are being made. If a doctor is going to rely on an ML algorithm to make a diagnosis or recommend a treatment, they need to know how that algorithm arrived at its conclusion.
The problem is, many ML models — particularly deep learning models — are essentially black boxes. They take in a bunch of data, do some magic math, and spit out a result. But understanding how they got from A to B is often impossible, even for the people who build them. That lack of interpretability is a huge barrier to trust and accountability in healthcare ML.
There are some promising approaches to this problem, like using attention mechanisms or building inherently interpretable models. But it’s still an active area of research, and there’s a lot of work to be done.
Scalability and performance
Finally, there are the nuts-and-bolts challenges of actually deploying ML systems in healthcare. Healthcare data is big — like, petabytes big. Processing and storing all that data requires serious computational horsepower and storage capacity. And in many cases, ML algorithms need to run in real-time to support clinical decision-making.
That means building infrastructure that can handle the scale and performance requirements of healthcare ML — optimizing algorithms for speed and efficiency, and finding ways to parallelize and distribute computation.
Ethical Concerns
Medical records, genetic data, behavioral data, even social media data. In the wrong hands, it could be used to discriminate, manipulate, or exploit people in ways we can barely imagine.
The risk of data breaches and unauthorized access is a huge concern for everyone working with machine learning models for healthcare. We’ve already seen high-profile cases of healthcare data being hacked or leaked, exposing millions of patients’ personal information. And as ML systems become more complex and interconnected, the attack surface only grows larger.
“Well-established companies mostly have data security covered. However, when we get addressed by startups we make it a rule to discuss data protection in the utmost detail to minimize the risks of all the parties involved — clients, product providers, and us, the developers.” — Amazinum
Informed consent and patient autonomy
But even when data is secure, there are still issues around consent and ownership.
- Do patients really understand what they’re agreeing to when they sign those HIPAA forms?
- Do they, entering clinical trials and research programs, know how their data might be used to train ML algorithms, or who might have access to it?
- And what about all the data that’s generated outside of clinical settings — the fitness trackers, the mental health apps, the online searches?
- Who owns that data, and what rights do patients have over it?
As ML systems become more sophisticated, they’re starting to make decisions that have real consequences for patients’ lives. A diagnostic algorithm might recommend a biopsy, or a treatment planning system might suggest a particular course of chemotherapy. These decisions are based on complex statistical models that even experts can struggle to understand.
Respecting patient autonomy means more than just getting a signature on a form. It means engaging patients in meaningful dialogue, explaining the limitations and uncertainties of ML systems, and letting them make decisions based on their own values and preferences.
Liability and accountability
Of course, even with the best intentions, things can go wrong. An algorithm might miss a critical diagnosis, or recommend a treatment that turns out to be harmful. When that happens, who’s liable? Is it the doctor who relied on the algorithm, the hospital that deployed it, or the company that developed it?
The truth is, our legal and regulatory frameworks are woefully unprepared for the problems related to the use of machine learning in healthcare. We’re still operating under a system that was designed for a world where medical decisions were made solely by human doctors, not machines. We need to update our laws and policies to reflect the new reality where AI tools play a critical role in healthcare.
That means establishing clear standards for the development, testing, and deployment of ML systems in healthcare.
“We encourage clients to invest in creating transparent and auditable systems that can be held accountable when things go wrong. Often it requires rethinking liability frameworks to ensure that everyone involved in the ML pipeline -— from data collectors to model developers to end users — has the right incentives to prioritize patient safety and wellbeing.” — Amazinum.
Equity and access
Finally, we need to deal with the hard reality that ML is not a panacea for all of healthcare’s ills. In fact, if we’re not careful, it could actually make some of those ills worse. One of the biggest risks is that ML could strengthen existing health disparities by reinforcing biases and inequities in the data and models we use.
We’ve already seen examples of this in other domains, like criminal justice and hiring, where ML systems have been shown to discriminate against certain groups based on race, gender, or other protected characteristics. We can’t let that happen in healthcare. We need to be proactive in ensuring that our ML systems are fair, equitable, and inclusive.
Collecting diverse and representative data sets, and using techniques like adversarial debiasing to mitigate bias in our models is a must.
We need to engage with communities that have been historically marginalized and excluded from healthcare, to understand their needs and build systems that work for them.
Healthcare is quite literally a matter of life and death.
If we’re going to use AI and ML to improve outcomes and save lives, we need to do it right.
- Application of Machine Learning: Why Healthcare? Why Now?
- Artificial Intelligence and Machine Learning for Healthcare Most Pressing Needs
- Who Can Make Those Machine Learning Algorithms Work for You?
- Machine Learning and Artificial Intelligence Tools in Healthcare
- Is There Something Left to Develop? Oh Yes, There Is
- Technical Challenges of Machine Learning in the Healthcare Industry
- Ethical Concerns
Planning to improve development process?
Decrease the entropy of product design and development, and increase your chances of success.
Written by
We Help With
Your tech partner needs to be well versed in all kinds of software-related services. As the software development process involves different stages and cycles, the most natural solution is to have them all performed by the same team of experts. That’s exactly what our diverse range of services is for.
The choice of technology for your software project is one of the defining factors of its success. Here at QArea, we have hands-on experience with dozens of popular front-end, back-end, and mobile technologies for creating robust software solutions.
In-depth familiarity and practical experience with key technologies are one of the cornerstones of successful software development and QA. But it also takes specific knowledge of the industry to develop a solution that meets the expectations of the stakeholders and propels its owner to success.

Ensure an effective online presence for your business with a corporate site.